LAPSE:2023.7066
Published Article
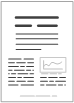
LAPSE:2023.7066
Deep Learning for Modeling an Offshore Hybrid Wind−Wave Energy System
February 24, 2023
The combination of an offshore wind turbine and a wave energy converter on an integrated platform is an economical solution for the electrical power demand in coastal countries. Due to the expensive installation cost, a prediction should be used to investigate whether the location is suitable for these sites. For this purpose, this research presents the feasibility of installing a combined hybrid site in the desired coastal location by predicting the net produced power due to the environmental parameters. For combining these two systems, an optimized array includes ten turbines and ten wave energy converters. The mathematical equations of the net force on the two introduced systems and the produced power of the wind turbines are proposed. The turbines’ maximum forces are 4 kN, and for the wave energy converters are 6 kN, respectively. Furthermore, the comparison is conducted in order to find the optimum system. The comparison shows that the most effective system of desired environmental condition is introduced. A number of machine learning and deep learning methods are used to predict key parameters after collecting the dataset. Moreover, a comparative analysis is conducted to find a suitable model. The models’ performance has been well studied through generating the confusion matrix and the receiver operating characteristic (ROC) curve of the hybrid site. The deep learning model outperformed other models, with an approximate accuracy of 0.96.
Record ID
Keywords
Artificial Intelligence, Big Data, comparative analysis, deep learning, Energy, Machine Learning, offshore, Renewable and Sustainable Energy, Wave Energy, wave power, wind turbine
Subject
Suggested Citation
Dehghan Manshadi M, Mousavi M, Soltani M, Mosavi A, Kovacs L. Deep Learning for Modeling an Offshore Hybrid Wind−Wave Energy System. (2023). LAPSE:2023.7066
Author Affiliations
Dehghan Manshadi M: Department of Mechanical Engineering, K. N. Toosi University of Technology, Tehran 1999143344, Iran
Mousavi M: Department of Mechanical Engineering, K. N. Toosi University of Technology, Tehran 1999143344, Iran; Faculty of Informatics, Selye University, 94501 Komarom, Slovakia [ORCID]
Soltani M: Department of Mechanical Engineering, K. N. Toosi University of Technology, Tehran 1999143344, Iran; Department of Electrical and Computer Engineering, University of Waterloo, Waterloo, ON N2L 3G1, Canada; Waterloo Institute for Sustainable Energy (WISE), [ORCID]
Mosavi A: German Research Center for Artificial Intelligence, 26129 Oldenburg, Germany; Institute of Information Engineering, Automation and Mathematics, Slovak University of Technology in Bratislava, 81243 Bratislava, Slovakia [ORCID]
Kovacs L: Biomatics and Applied Artificial Intelligence Institution, John von Neumann Faculty of Informatics, Obuda University, 1034 Budapest, Hungary; Physiological Controls Research Center, Obuda University, 1034 Budapest, Hungary [ORCID]
Mousavi M: Department of Mechanical Engineering, K. N. Toosi University of Technology, Tehran 1999143344, Iran; Faculty of Informatics, Selye University, 94501 Komarom, Slovakia [ORCID]
Soltani M: Department of Mechanical Engineering, K. N. Toosi University of Technology, Tehran 1999143344, Iran; Department of Electrical and Computer Engineering, University of Waterloo, Waterloo, ON N2L 3G1, Canada; Waterloo Institute for Sustainable Energy (WISE), [ORCID]
Mosavi A: German Research Center for Artificial Intelligence, 26129 Oldenburg, Germany; Institute of Information Engineering, Automation and Mathematics, Slovak University of Technology in Bratislava, 81243 Bratislava, Slovakia [ORCID]
Kovacs L: Biomatics and Applied Artificial Intelligence Institution, John von Neumann Faculty of Informatics, Obuda University, 1034 Budapest, Hungary; Physiological Controls Research Center, Obuda University, 1034 Budapest, Hungary [ORCID]
Journal Name
Energies
Volume
15
Issue
24
First Page
9484
Year
2022
Publication Date
2022-12-14
Published Version
ISSN
1996-1073
Version Comments
Original Submission
Other Meta
PII: en15249484, Publication Type: Journal Article
Record Map
Published Article
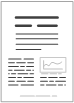
LAPSE:2023.7066
This Record
External Link
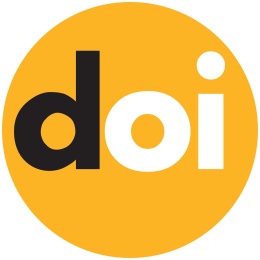
doi:10.3390/en15249484
Publisher Version
Download
Meta
Record Statistics
Record Views
75
Version History
[v1] (Original Submission)
Feb 24, 2023
Verified by curator on
Feb 24, 2023
This Version Number
v1
Citations
Most Recent
This Version
URL Here
https://psecommunity.org/LAPSE:2023.7066
Original Submitter
Auto Uploader for LAPSE
Links to Related Works