LAPSE:2023.32782
Published Article
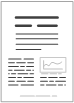
LAPSE:2023.32782
Deep Convolutional Feature-Based Probabilistic SVDD Method for Monitoring Incipient Faults of Batch Process
April 20, 2023
Support vector data description (SVDD) has been widely applied to batch process fault detection. However, it often performs poorly, especially when incipient faults occur, because it only considers the shallow data feature and omits the probabilistic information of features. In order to provide better monitoring performance on incipient faults in batch processes, an improved SVDD method, called deep probabilistic SVDD (DPSVDD), is proposed in this work by integrating the convolutional autoencoder and the probability-related monitoring indices. For mining the hidden data features effectively, a deep convolutional features extraction network is designed by a convolutional autoencoder, where the encoder outputs and the reconstruction errors are used as the monitor features. Furthermore, the probability distribution changes of these features are evaluated by the Kullback-Leibler (KL) divergence so that the probability-related monitoring indices are developed for indicating the process status. The applications to the benchmark penicillin fermentation process demonstrate that the proposed method has a better monitoring performance on the incipient faults in comparison to the traditional SVDD methods.
Record ID
Keywords
Batch Process, deep learning, incipient fault, support vector data description
Subject
Suggested Citation
Wang X, Wang Y, Deng X, Zhang Z. Deep Convolutional Feature-Based Probabilistic SVDD Method for Monitoring Incipient Faults of Batch Process. (2023). LAPSE:2023.32782
Author Affiliations
Wang X: College of Control Science and Engineering, China University of Petroleum, Qingdao 266580, China; College of Application Technology, Qingdao University, Qingdao 266071, China
Wang Y: College of Control Science and Engineering, China University of Petroleum, Qingdao 266580, China
Deng X: College of Control Science and Engineering, China University of Petroleum, Qingdao 266580, China [ORCID]
Zhang Z: College of Control Science and Engineering, China University of Petroleum, Qingdao 266580, China
Wang Y: College of Control Science and Engineering, China University of Petroleum, Qingdao 266580, China
Deng X: College of Control Science and Engineering, China University of Petroleum, Qingdao 266580, China [ORCID]
Zhang Z: College of Control Science and Engineering, China University of Petroleum, Qingdao 266580, China
Journal Name
Energies
Volume
14
Issue
11
First Page
3334
Year
2021
Publication Date
2021-06-06
Published Version
ISSN
1996-1073
Version Comments
Original Submission
Other Meta
PII: en14113334, Publication Type: Journal Article
Record Map
Published Article
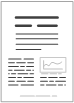
LAPSE:2023.32782
This Record
External Link
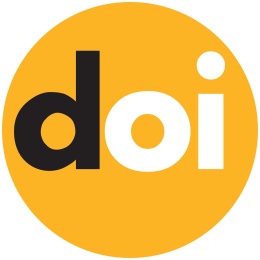
doi:10.3390/en14113334
Publisher Version
Download
Meta
Record Statistics
Record Views
114
Version History
[v1] (Original Submission)
Apr 20, 2023
Verified by curator on
Apr 20, 2023
This Version Number
v1
Citations
Most Recent
This Version
URL Here
https://psecommunity.org/LAPSE:2023.32782
Original Submitter
Auto Uploader for LAPSE
Links to Related Works