LAPSE:2023.27972
Published Article
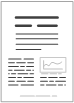
LAPSE:2023.27972
Reinforcement Learning-Based School Energy Management System
April 11, 2023
Energy efficiency is a key to reduced carbon footprint, savings on energy bills, and sustainability for future generations. For instance, in hot climate countries such as Qatar, buildings are high energy consumers due to air conditioning that resulted from high temperatures and humidity. Optimizing the building energy management system will reduce unnecessary energy consumptions, improve indoor environmental conditions, maximize building occupant’s comfort, and limit building greenhouse gas emissions. However, lowering energy consumption cannot be done despite the occupants’ comfort. Solutions must take into account these tradeoffs. Conventional Building Energy Management methods suffer from a high dimensional and complex control environment. In recent years, the Deep Reinforcement Learning algorithm, applying neural networks for function approximation, shows promising results in handling such complex problems. In this work, a Deep Reinforcement Learning agent is proposed for controlling and optimizing a school building’s energy consumption. It is designed to search for optimal policies to minimize energy consumption, maintain thermal comfort, and reduce indoor contaminant levels in a challenging 21-zone environment. First, the agent is trained with the baseline in a supervised learning framework. After cloning the baseline strategy, the agent learns with proximal policy optimization in an actor-critic framework. The performance is evaluated on a school model simulated environment considering thermal comfort, CO2 levels, and energy consumption. The proposed methodology can achieve a 21% reduction in energy consumption, a 44% better thermal comfort, and healthier CO2 concentrations over a one-year simulation, with reduced training time thanks to the integration of the behavior cloning learning technique.
Record ID
Keywords
Energy Efficiency, energy management, indoor air quality, reinforcement learning, smart building, thermal comfort
Subject
Suggested Citation
Chemingui Y, Gastli A, Ellabban O. Reinforcement Learning-Based School Energy Management System. (2023). LAPSE:2023.27972
Author Affiliations
Chemingui Y: Electrical Engineering Department, Qatar University, P.O. Box 2713 Doha, Qatar [ORCID]
Gastli A: Electrical Engineering Department, Qatar University, P.O. Box 2713 Doha, Qatar [ORCID]
Ellabban O: Iberdrola Innovation Middle East, Qatar Science & Technology Park, P.O. Box 210177 Doha, Qatar [ORCID]
Gastli A: Electrical Engineering Department, Qatar University, P.O. Box 2713 Doha, Qatar [ORCID]
Ellabban O: Iberdrola Innovation Middle East, Qatar Science & Technology Park, P.O. Box 210177 Doha, Qatar [ORCID]
Journal Name
Energies
Volume
13
Issue
23
Article Number
E6354
Year
2020
Publication Date
2020-12-01
Published Version
ISSN
1996-1073
Version Comments
Original Submission
Other Meta
PII: en13236354, Publication Type: Journal Article
Record Map
Published Article
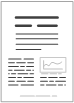
LAPSE:2023.27972
This Record
External Link
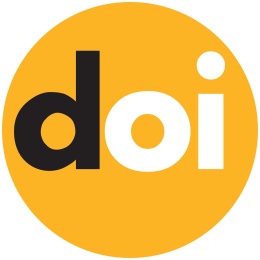
doi:10.3390/en13236354
Publisher Version
Download
Meta
Record Statistics
Record Views
56
Version History
[v1] (Original Submission)
Apr 11, 2023
Verified by curator on
Apr 11, 2023
This Version Number
v1
Citations
Most Recent
This Version
URL Here
https://psecommunity.org/LAPSE:2023.27972
Original Submitter
Auto Uploader for LAPSE
Links to Related Works