LAPSE:2023.26565
Published Article
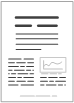
LAPSE:2023.26565
An Online Energy-Saving Driving Strategy for Metro Train Operation Based on the Model Predictive Control of Switched-Mode Dynamical Systems
April 3, 2023
With the rapid development of urban rail transit systems and the consequent sharp increase of energy consumption, the energy-saving train operation problem has been attracting much attention. Extensive studies have been devoted to optimal control of a single metro train in an inter-station run to minimize the energy consumption. However, most of the existing work focuses on offline optimization of the energy-saving driving strategy, which still needs to be tracked in real train operation. In order to attain better performance in the presence of disturbances, this paper studies the online optimization problem of the energy-saving driving strategy for a single metro train, by employing the model predictive control (MPC) approach. Firstly, a switched-mode dynamical system model is introduced to describe the dynamics of a metro train. Based on this model, an MPC-based online optimization problem is formulated for obtaining the optimal mode switching times with minimal energy consumption for a single train in an inter-station run. Then we propose an algorithm to solve the constrained optimization problem at each time step by utilizing the exterior point penalty function method. The proposed online optimal train control algorithm which determines the mode switching times can not only improve the computational efficiency but also enhances the robustness to disturbances in real scenarios. Finally, the effectiveness and advantages of this online optimal train control algorithm are illustrated through case studies of a single train in an inter-station run.
Record ID
Keywords
energy saving, metro train, Model Predictive Control, online, switched-mode dynamical systems
Subject
Suggested Citation
Shang F, Zhan J, Chen Y. An Online Energy-Saving Driving Strategy for Metro Train Operation Based on the Model Predictive Control of Switched-Mode Dynamical Systems. (2023). LAPSE:2023.26565
Author Affiliations
Shang F: Beijing Key Laboratory of Traffic Engineering, Beijing University of Technology, Beijing 100124, China [ORCID]
Zhan J: College of Artificial Intelligence and Automation, Beijing University of Technology, Beijing 100124, China
Chen Y: College of Artificial Intelligence and Automation, Beijing University of Technology, Beijing 100124, China
Zhan J: College of Artificial Intelligence and Automation, Beijing University of Technology, Beijing 100124, China
Chen Y: College of Artificial Intelligence and Automation, Beijing University of Technology, Beijing 100124, China
Journal Name
Energies
Volume
13
Issue
18
Article Number
E4933
Year
2020
Publication Date
2020-09-20
Published Version
ISSN
1996-1073
Version Comments
Original Submission
Other Meta
PII: en13184933, Publication Type: Journal Article
Record Map
Published Article
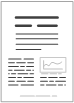
LAPSE:2023.26565
This Record
External Link
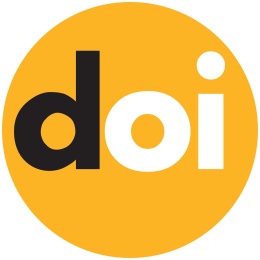
doi:10.3390/en13184933
Publisher Version
Download
Meta
Record Statistics
Record Views
84
Version History
[v1] (Original Submission)
Apr 3, 2023
Verified by curator on
Apr 3, 2023
This Version Number
v1
Citations
Most Recent
This Version
URL Here
https://psecommunity.org/LAPSE:2023.26565
Original Submitter
Auto Uploader for LAPSE
Links to Related Works