LAPSE:2023.18906
Published Article
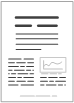
LAPSE:2023.18906
A Pattern New in Every Moment: The Temporal Clustering of Markets for Crude Oil, Refined Fuels, and Other Commodities
March 9, 2023
The identification of critical periods and business cycles contributes significantly to the analysis of financial markets and the macroeconomy. Financialization and cointegration place a premium on the accurate recognition of time-varying volatility in commodity markets, especially those for crude oil and refined fuels. This article seeks to identify critical periods in the trading of energy-related commodities as a step toward understanding the temporal dynamics of those markets. This article proposes a novel application of unsupervised machine learning. A suite of clustering methods, applied to conditional volatility forecasts by trading days and individual assets or asset classes, can identify critical periods in energy-related commodity markets. Unsupervised machine learning achieves this task without rules-based or subjective definitions of crises. Five clustering methods—affinity propagation, mean-shift, spectral, k-means, and hierarchical agglomerative clustering—can identify anomalous periods in commodities trading. These methods identified the financial crisis of 2008−2009 and the initial stages of the COVID-19 pandemic. Applied to four energy-related markets—Brent, West Texas intermediate, gasoil, and gasoline—the same methods identified additional periods connected to events such as the September 11 terrorist attacks and the 2003 Persian Gulf war. t-distributed stochastic neighbor embedding facilitates the visualization of trading regimes. Temporal clustering of conditional volatility forecasts reveals unusual financial properties that distinguish the trading of energy-related commodities during critical periods from trading during normal periods and from trade in other commodities in all periods. Whereas critical periods for all commodities appear to coincide with broader disruptions in demand for energy, critical periods unique to crude oil and refined fuels appear to arise from acute disruptions in supply. Extensions of these methods include the definition of bull and bear markets and the identification of recessions and recoveries in the real economy.
Record ID
Keywords
Brent, clustering, COVID-19 pandemic, energy commodities, financial crises, Gasoline, Machine Learning, t-SNE, WTI
Subject
Suggested Citation
Chen JM, Rehman MU. A Pattern New in Every Moment: The Temporal Clustering of Markets for Crude Oil, Refined Fuels, and Other Commodities. (2023). LAPSE:2023.18906
Author Affiliations
Chen JM: College of Law, Michigan State University, East Lansing, MI 48824, USA [ORCID]
Rehman MU: Management Sciences, Shaheed Zulfikar Ali Bhutto Institute of Science and Technology (SZABIST), Islamabad 44000, Pakistan
Rehman MU: Management Sciences, Shaheed Zulfikar Ali Bhutto Institute of Science and Technology (SZABIST), Islamabad 44000, Pakistan
Journal Name
Energies
Volume
14
Issue
19
First Page
6099
Year
2021
Publication Date
2021-09-24
Published Version
ISSN
1996-1073
Version Comments
Original Submission
Other Meta
PII: en14196099, Publication Type: Journal Article
Record Map
Published Article
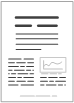
LAPSE:2023.18906
This Record
External Link
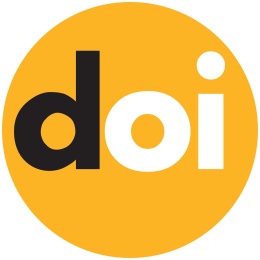
doi:10.3390/en14196099
Publisher Version
Download
Meta
Record Statistics
Record Views
123
Version History
[v1] (Original Submission)
Mar 9, 2023
Verified by curator on
Mar 9, 2023
This Version Number
v1
Citations
Most Recent
This Version
URL Here
https://psecommunity.org/LAPSE:2023.18906
Original Submitter
Auto Uploader for LAPSE
Links to Related Works