LAPSE:2023.1057
Published Article
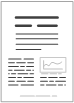
LAPSE:2023.1057
Comparative Performance of Machine-Learning and Deep-Learning Algorithms in Predicting Gas−Liquid Flow Regimes
February 21, 2023
Gas−liquid flow is a significant phenomenon in various engineering applications, such as in nuclear reactors, power plants, chemical industries, and petroleum industries. The prediction of the flow patterns is of great importance for designing and analyzing the operations of two-phase pipeline systems. The traditional numerical and empirical methods that have been used for the prediction are known to result in a high inaccuracy for scale-up processes. That is why various artificial intelligence-based (AI-based) methodologies are being applied, at present, to predict the gas−liquid flow regimes. We focused in the current study on a thorough comparative analysis of machine learning (ML) and deep learning (DL) in predicting the flow regimes with the application of a diverse set of ML and DL frameworks to a database comprising 11,837 data points, which were collected from thirteen independent experiments. During the pre-processing, the big data analysis was performed to analyze the correlations among the parameters and extract important features. The comparative analysis of the AI-based models’ performances was conducted using precision, recall, F1-score, accuracy, Cohen’s kappa, and receiver operating characteristics curves. The extreme gradient boosting method was identified as the optimum model for predicting the two-phase flow regimes in inclined or horizontal pipelines.
Record ID
Keywords
Artificial Intelligence, Modelling, multiphase flow, pipeline, prediction
Subject
Suggested Citation
Hafsa N, Rushd S, Yousuf H. Comparative Performance of Machine-Learning and Deep-Learning Algorithms in Predicting Gas−Liquid Flow Regimes. (2023). LAPSE:2023.1057
Author Affiliations
Hafsa N: Department of Computer Science, College of Computer Science and Information Technology, King Faisal University, Al-Ahsa 31982, Saudi Arabia [ORCID]
Rushd S: Department of Chemical Engineering, College of Engineering, King Faisal University, Al-Ahsa 31982, Saudi Arabia [ORCID]
Yousuf H: Department of Petroleum and Mineral Resources Engineering, Bangladesh University of Engineering and Technology, Dhaka 1000, Bangladesh
Rushd S: Department of Chemical Engineering, College of Engineering, King Faisal University, Al-Ahsa 31982, Saudi Arabia [ORCID]
Yousuf H: Department of Petroleum and Mineral Resources Engineering, Bangladesh University of Engineering and Technology, Dhaka 1000, Bangladesh
Journal Name
Processes
Volume
11
Issue
1
First Page
177
Year
2023
Publication Date
2023-01-06
Published Version
ISSN
2227-9717
Version Comments
Original Submission
Other Meta
PII: pr11010177, Publication Type: Journal Article
Record Map
Published Article
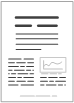
LAPSE:2023.1057
This Record
External Link
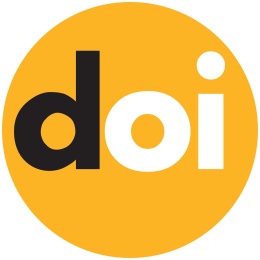
doi:10.3390/pr11010177
Publisher Version
Download
Meta
Record Statistics
Record Views
94
Version History
[v1] (Original Submission)
Feb 21, 2023
Verified by curator on
Feb 21, 2023
This Version Number
v1
Citations
Most Recent
This Version
URL Here
https://psecommunity.org/LAPSE:2023.1057
Original Submitter
Auto Uploader for LAPSE
Links to Related Works