LAPSE:2024.0263
Published Article
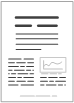
LAPSE:2024.0263
Machine Learning Aided Prediction of Glass-Forming Ability of Metallic Glass
February 19, 2024
The prediction of the glass-forming ability (GFA) of metallic glasses (MGs) can accelerate the efficiency of their development. In this paper, a dataset was constructed using experimental data collected from the literature and books, and a machine learning-based predictive model was established to predict the GFA. Firstly, a classification model based on the size of the critical diameter (Dmax) was established to determine whether an alloy system could form a glass state, with an accuracy rating of 0.98. Then, regression models were established to predict the crystallization temperature (Tx), glass transition temperature (Tg), and liquidus temperature (Tl) of MGs. The R2 of the prediction model obtained in the test set was greater than 0.89, which showed that the model had good prediction accuracy. The key features used by the regression models were analyzed using variance, correlation, embedding, recursive, and exhaustive methods to select the most important features. Furthermore, to improve the interpretability of the prediction model, feature importance, partial dependence plot (PDP), and individual conditional expectation (ICE) methods were used for visualization analysis, demonstrating how features affect the target variables. Finally, taking Zr-Cu-Ni-Al system MGs as an example, a prediction model was established using a genetic algorithm to optimize the alloy composition for high GFA in the compositional space, achieving the optimal design of alloy composition.
Record ID
Keywords
glass-forming ability, Machine Learning, metallic glass, optimal design
Subject
Suggested Citation
Liu C, Wang X, Cai W, He Y, Su H. Machine Learning Aided Prediction of Glass-Forming Ability of Metallic Glass. (2024). LAPSE:2024.0263
Author Affiliations
Liu C: Institute of Structural Steel, Central Iron and Steel Research Institute, Beijing 100081, China; Material Digital R&D Center, China Iron and Steel Research Institute Group, Beijing 100081, China
Wang X: Material Digital R&D Center, China Iron and Steel Research Institute Group, Beijing 100081, China
Cai W: Material Digital R&D Center, China Iron and Steel Research Institute Group, Beijing 100081, China
He Y: Material Digital R&D Center, China Iron and Steel Research Institute Group, Beijing 100081, China
Su H: Material Digital R&D Center, China Iron and Steel Research Institute Group, Beijing 100081, China
Wang X: Material Digital R&D Center, China Iron and Steel Research Institute Group, Beijing 100081, China
Cai W: Material Digital R&D Center, China Iron and Steel Research Institute Group, Beijing 100081, China
He Y: Material Digital R&D Center, China Iron and Steel Research Institute Group, Beijing 100081, China
Su H: Material Digital R&D Center, China Iron and Steel Research Institute Group, Beijing 100081, China
Journal Name
Processes
Volume
11
Issue
9
First Page
2806
Year
2023
Publication Date
2023-09-21
Published Version
ISSN
2227-9717
Version Comments
Original Submission
Other Meta
PII: pr11092806, Publication Type: Journal Article
Record Map
Published Article
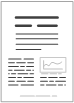
LAPSE:2024.0263
This Record
External Link
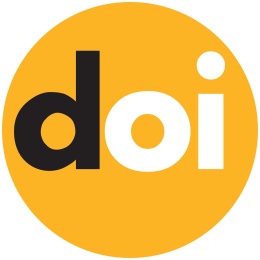
doi:10.3390/pr11092806
Publisher Version
Download
Meta
Record Statistics
Record Views
49
Version History
[v1] (Original Submission)
Feb 19, 2024
Verified by curator on
Feb 19, 2024
This Version Number
v1
Citations
Most Recent
This Version
URL Here
https://psecommunity.org/LAPSE:2024.0263
Original Submitter
Calvin Tsay
Links to Related Works