LAPSE:2024.0208
Published Article
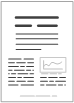
LAPSE:2024.0208
Design and Implementation of Defect Detection System Based on YOLOv5-CBAM for Lead Tabs in Secondary Battery Manufacturing
February 10, 2024
According to QYResearch, a global market research firm, the global market size of secondary batteries is growing at an average annual rate of 8.1%, but fires and casualties continue to occur due to the lack of quality and reliability of secondary batteries. Therefore, improving the quality of secondary batteries is a major factor in determining a company’s competitive advantage. In particular, lead taps, which electrically connect the negative and positive electrodes of secondary batteries, are a key factor in determining the stability of the battery. Currently, the quality inspection of secondary battery lead tab manufacturers mostly consists of visual inspection after vision inspection with a rule-based algorithm, which has limitations on the types of defects that can be detected, and the inspection time is increasing due to overlapping inspections, which is directly related to productivity. Therefore, this study aims to automate the quality inspection of lead tabs of secondary batteries by applying deep-learning-based algorithms to improve inspection accuracy, improve reliability, and improve productivity. We selected the YOLOv5 model, which, among deep-learning algorithms, has a benefit for object detection, and used the YOLOv5_CBAM model, which replaces the bottleneck part in the C3 layer of YOLOv5 with the Convolutional Block Attention Module (CBAM) based on the attention mechanism, to improve the accuracy and speed of the model. As a result of applying the YOLOv5_CBAM model, we found that the parameter was reduced by more than 50% and the performance was improved by 2%. In addition, image processing was applied to help segment the defective area to apply the SPEC value for each defective object after detection.
Record ID
Keywords
automatic defect detection, CBAM, computer vision, deep learning, lead tap, object detection, YOLOv5
Subject
Suggested Citation
Mun J, Kim J, Do Y, Kim H, Lee C, Jeong J. Design and Implementation of Defect Detection System Based on YOLOv5-CBAM for Lead Tabs in Secondary Battery Manufacturing. (2024). LAPSE:2024.0208
Author Affiliations
Mun J: Department of Smart Factory Convergence, Sungkyunkwan University, 2066 Seobu-ro, Jangan-gu, Suwon 16419, Republic of Korea; Hygino AI Research Laboratory, 25, Simin-daero 248 Beon-gil, Dongan-gu, Anyang 14067, Republic of Korea [ORCID]
Kim J: Department of Smart Factory Convergence, Sungkyunkwan University, 2066 Seobu-ro, Jangan-gu, Suwon 16419, Republic of Korea [ORCID]
Do Y: Department of Smart Factory Convergence, Sungkyunkwan University, 2066 Seobu-ro, Jangan-gu, Suwon 16419, Republic of Korea
Kim H: Hygino AI Research Laboratory, 25, Simin-daero 248 Beon-gil, Dongan-gu, Anyang 14067, Republic of Korea
Lee C: Department of Smart Factory Convergence, Sungkyunkwan University, 2066 Seobu-ro, Jangan-gu, Suwon 16419, Republic of Korea
Jeong J: Department of Smart Factory Convergence, Sungkyunkwan University, 2066 Seobu-ro, Jangan-gu, Suwon 16419, Republic of Korea [ORCID]
Kim J: Department of Smart Factory Convergence, Sungkyunkwan University, 2066 Seobu-ro, Jangan-gu, Suwon 16419, Republic of Korea [ORCID]
Do Y: Department of Smart Factory Convergence, Sungkyunkwan University, 2066 Seobu-ro, Jangan-gu, Suwon 16419, Republic of Korea
Kim H: Hygino AI Research Laboratory, 25, Simin-daero 248 Beon-gil, Dongan-gu, Anyang 14067, Republic of Korea
Lee C: Department of Smart Factory Convergence, Sungkyunkwan University, 2066 Seobu-ro, Jangan-gu, Suwon 16419, Republic of Korea
Jeong J: Department of Smart Factory Convergence, Sungkyunkwan University, 2066 Seobu-ro, Jangan-gu, Suwon 16419, Republic of Korea [ORCID]
Journal Name
Processes
Volume
11
Issue
9
First Page
2751
Year
2023
Publication Date
2023-09-14
Published Version
ISSN
2227-9717
Version Comments
Original Submission
Other Meta
PII: pr11092751, Publication Type: Journal Article
Record Map
Published Article
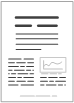
LAPSE:2024.0208
This Record
External Link
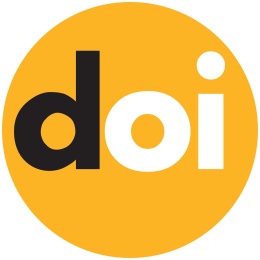
doi:10.3390/pr11092751
Publisher Version
Download
Meta
Record Statistics
Record Views
43
Version History
[v1] (Original Submission)
Feb 10, 2024
Verified by curator on
Feb 10, 2024
This Version Number
v1
Citations
Most Recent
This Version
URL Here
https://psecommunity.org/LAPSE:2024.0208
Original Submitter
Calvin Tsay
Links to Related Works