LAPSE:2024.0195
Published Article
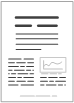
LAPSE:2024.0195
Research on a Carbon Emission Prediction Method for Oil Field Transfer Stations Based on an Improved Genetic Algorithm—The Decision Tree Algorithm
February 10, 2024
The background of “dual carbon” is accelerating low-carbon transformation in the energy field, and oil field enterprises are facing challenges in energy conservation and emissions reduction for sustainable development. However, oil field gathering and transfer station systems, which are crucial components of the onshore transportation system, face challenges in energy conservation and emissions reduction. Therefore, it is necessary to predict the carbon emissions of oil field gathering and transfer station systems. To improve the accuracy of carbon emission prediction for the system, this study proposes an improved GA-decision tree (IGA-decision tree) algorithm. First, chaotic mapping was introduced to initialize the population, ensuring a uniform distribution of initial particles in the search space and enhancing population diversity. Second, the firefly perturbation strategy was employed to avoid the problem of genetic algorithms becoming trapped in local optima during the later stages of the search. The results show that the enhanced GA-decision tree algorithm effectively avoided being stuck in local optima while performing global searches. When predicting the carbon emissions of oil field gathering and transfer stations, the improved GA-decision tree (IGA-decision tree) algorithm outperformed traditional decision tree and GA-decision tree algorithms in terms of error and convergence efficiency. It achieved a root mean square error (RMSE) value of 74.5181 and a correlation coefficient (R2) of 0.99, indicating a high level of fitness and good convergence, as well as high prediction accuracy. This algorithm contributes to carbon accounting and energy conservation efforts in oilfield gathering and the transfer station system, filling the research gap in carbon emissions prediction for the system within the framework of energy internet projects.
Record ID
Keywords
“dual carbon”, carbon emission forecasting, energy saving and emissions reduction, IGA-decision tree algorithm, transfer station system
Subject
Suggested Citation
Cheng Q, Wang X, Wang S, Li Y, Liu H, Li Z, Sun W. Research on a Carbon Emission Prediction Method for Oil Field Transfer Stations Based on an Improved Genetic Algorithm—The Decision Tree Algorithm. (2024). LAPSE:2024.0195
Author Affiliations
Cheng Q: Key Lab of Ministry of Education for Enhancing the Oil and Gas Recovery Ratio, Northeast Petroleum University, Daqing 163318, China
Wang X: Key Lab of Ministry of Education for Enhancing the Oil and Gas Recovery Ratio, Northeast Petroleum University, Daqing 163318, China
Wang S: Key Lab of Ministry of Education for Enhancing the Oil and Gas Recovery Ratio, Northeast Petroleum University, Daqing 163318, China
Li Y: Key Lab of Ministry of Education for Enhancing the Oil and Gas Recovery Ratio, Northeast Petroleum University, Daqing 163318, China
Liu H: Key Lab of Ministry of Education for Enhancing the Oil and Gas Recovery Ratio, Northeast Petroleum University, Daqing 163318, China
Li Z: Key Lab of Ministry of Education for Enhancing the Oil and Gas Recovery Ratio, Northeast Petroleum University, Daqing 163318, China
Sun W: Key Lab of Ministry of Education for Enhancing the Oil and Gas Recovery Ratio, Northeast Petroleum University, Daqing 163318, China
Wang X: Key Lab of Ministry of Education for Enhancing the Oil and Gas Recovery Ratio, Northeast Petroleum University, Daqing 163318, China
Wang S: Key Lab of Ministry of Education for Enhancing the Oil and Gas Recovery Ratio, Northeast Petroleum University, Daqing 163318, China
Li Y: Key Lab of Ministry of Education for Enhancing the Oil and Gas Recovery Ratio, Northeast Petroleum University, Daqing 163318, China
Liu H: Key Lab of Ministry of Education for Enhancing the Oil and Gas Recovery Ratio, Northeast Petroleum University, Daqing 163318, China
Li Z: Key Lab of Ministry of Education for Enhancing the Oil and Gas Recovery Ratio, Northeast Petroleum University, Daqing 163318, China
Sun W: Key Lab of Ministry of Education for Enhancing the Oil and Gas Recovery Ratio, Northeast Petroleum University, Daqing 163318, China
Journal Name
Processes
Volume
11
Issue
9
First Page
2738
Year
2023
Publication Date
2023-09-14
Published Version
ISSN
2227-9717
Version Comments
Original Submission
Other Meta
PII: pr11092738, Publication Type: Journal Article
Record Map
Published Article
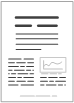
LAPSE:2024.0195
This Record
External Link
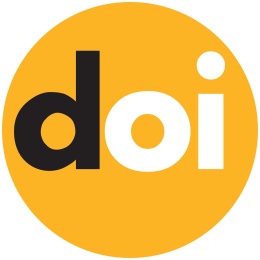
doi:10.3390/pr11092738
Publisher Version
Download
Meta
Record Statistics
Record Views
53
Version History
[v1] (Original Submission)
Feb 10, 2024
Verified by curator on
Feb 10, 2024
This Version Number
v1
Citations
Most Recent
This Version
URL Here
https://psecommunity.org/LAPSE:2024.0195
Original Submitter
Calvin Tsay
Links to Related Works