LAPSE:2023.8795
Published Article
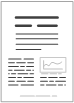
LAPSE:2023.8795
Power System Fault Diagnosis Method Based on Deep Reinforcement Learning
February 24, 2023
Intelligent power grid fault diagnosis is of great significance for speeding up fault processing and improving fault diagnosis efficiency. However, most of the current fault diagnosis methods focus on rule diagnosis, relying on expert experience and logical rules to build a diagnosis model, and lack the ability to automatically extract fault knowledge. For switch refusal events, it is difficult to determine a refusal switch without network topology. In order to realize the non-operating switch identification without network topology, this paper proposes a power grid fault diagnosis method based on deep reinforcement learning for alarm information text. Taking the single alarm information of the non-switch refusal sample as the research object, through the self-learning ability of deep reinforcement learning, it learns the topology connection relationship and action logic relationship between equipment, protection and circuit breakers contained in the alarm information, and realizes the detection of fault events. The correct prediction of the fault removal process after the occurrence, based on this, determines the refusal switch when the switch refuses to operate during the fault removal process. The calculation example results show that the proposed method can effectively diagnose the refusal switch of the switch refusal event, which is feasible and effective.
Record ID
Keywords
alarm information, deep Q-network, deep reinforcement learning, fault diagnosis
Subject
Suggested Citation
Wang Z, Zhang Z, Zhang X, Du M, Zhang H, Liu B. Power System Fault Diagnosis Method Based on Deep Reinforcement Learning. (2023). LAPSE:2023.8795
Author Affiliations
Wang Z: School of Electrical and Electronic Engineering, North China Electric Power University, Beijing 102206, China
Zhang Z: School of Electrical and Electronic Engineering, North China Electric Power University, Beijing 102206, China [ORCID]
Zhang X: School of Electrical and Electronic Engineering, North China Electric Power University, Beijing 102206, China
Du M: School of Electrical and Electronic Engineering, North China Electric Power University, Beijing 102206, China [ORCID]
Zhang H: State Grid Shanxi Electric Power Company Skills Training Center, Shanxi Electric Power Vocational and Technical Institute, Taiyuan 030021, China
Liu B: School of Electrical and Electronic Engineering, North China Electric Power University, Beijing 102206, China
Zhang Z: School of Electrical and Electronic Engineering, North China Electric Power University, Beijing 102206, China [ORCID]
Zhang X: School of Electrical and Electronic Engineering, North China Electric Power University, Beijing 102206, China
Du M: School of Electrical and Electronic Engineering, North China Electric Power University, Beijing 102206, China [ORCID]
Zhang H: State Grid Shanxi Electric Power Company Skills Training Center, Shanxi Electric Power Vocational and Technical Institute, Taiyuan 030021, China
Liu B: School of Electrical and Electronic Engineering, North China Electric Power University, Beijing 102206, China
Journal Name
Energies
Volume
15
Issue
20
First Page
7639
Year
2022
Publication Date
2022-10-16
Published Version
ISSN
1996-1073
Version Comments
Original Submission
Other Meta
PII: en15207639, Publication Type: Journal Article
Record Map
Published Article
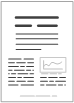
LAPSE:2023.8795
This Record
External Link
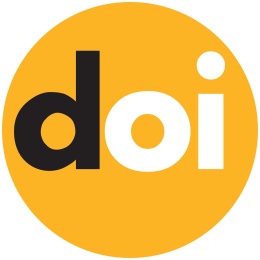
doi:10.3390/en15207639
Publisher Version
Download
Meta
Record Statistics
Record Views
37
Version History
[v1] (Original Submission)
Feb 24, 2023
Verified by curator on
Feb 24, 2023
This Version Number
v1
Citations
Most Recent
This Version
URL Here
https://psecommunity.org/LAPSE:2023.8795
Original Submitter
Auto Uploader for LAPSE
Links to Related Works