LAPSE:2023.7499
Published Article
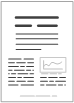
LAPSE:2023.7499
Application of LSTM Approach for Predicting the Fission Swelling Behavior within a CERCER Composite Fuel
February 24, 2023
Irradiation-induced swelling plays a key role in determining fuel performance. Due to their high cost and time demands, experimental research methods are ineffective. Knowledge-based multiscale simulations are also constrained by the loss of trustworthy theoretical underpinnings. This work presents a new trial of integrating knowledge-based finite element analysis (FEA) with a data-driven deep learning framework, to predict the hydrostatic-pressure−temperature dependent fission swelling behavior within a CERCER composite fuel. We employed the long short-term memory (LSTM) deep learning network to mimic the history-dependent behaviors. Training of the LSTM is achieved by processing the sequential order of the inputs to do the forecasting; the input features are fission rate, fission density, temperature, and hydrostatic pressure. We performed the model training based on a leveraged dataset of 8000 combinations of a wide range of input states and state evaluations that were generated by high-fidelity simulations. When replicating the swelling plots, the trained LSTM deep learning model exhibits outstanding prediction effectiveness. For various input variables, the model successfully pinpoints when recrystallization first occurs. The preliminary study for model interpretation suggests providing quantified insights into how those features affect solid and gaseous portions of swelling. The study demonstrates the efficacy of combining data-driven and knowledge-based modeling techniques to assess irradiation-induced fuel performance and enhance future design.
Record ID
Keywords
data-driven, finite element analysis, fission swelling, LSTM deep learning, Multiscale Modelling
Subject
Suggested Citation
Zhao J, Chen Z, Tu J, Zhao Y, Dong Y. Application of LSTM Approach for Predicting the Fission Swelling Behavior within a CERCER Composite Fuel. (2023). LAPSE:2023.7499
Author Affiliations
Zhao J: School of Aerospace Engineering and Applied Mechanics, Tongji University, Shanghai 200092, China
Chen Z: School of Aerospace Engineering and Applied Mechanics, Tongji University, Shanghai 200092, China
Tu J: School of Aerospace Engineering and Applied Mechanics, Tongji University, Shanghai 200092, China; Department of Aeronautics and Astronautics, Fudan University, Shanghai 200433, China
Zhao Y: School of Aerospace Engineering and Applied Mechanics, Tongji University, Shanghai 200092, China [ORCID]
Dong Y: Department of Aeronautics and Astronautics, Fudan University, Shanghai 200433, China
Chen Z: School of Aerospace Engineering and Applied Mechanics, Tongji University, Shanghai 200092, China
Tu J: School of Aerospace Engineering and Applied Mechanics, Tongji University, Shanghai 200092, China; Department of Aeronautics and Astronautics, Fudan University, Shanghai 200433, China
Zhao Y: School of Aerospace Engineering and Applied Mechanics, Tongji University, Shanghai 200092, China [ORCID]
Dong Y: Department of Aeronautics and Astronautics, Fudan University, Shanghai 200433, China
Journal Name
Energies
Volume
15
Issue
23
First Page
9053
Year
2022
Publication Date
2022-11-29
Published Version
ISSN
1996-1073
Version Comments
Original Submission
Other Meta
PII: en15239053, Publication Type: Journal Article
Record Map
Published Article
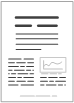
LAPSE:2023.7499
This Record
External Link
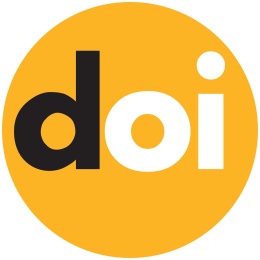
doi:10.3390/en15239053
Publisher Version
Download
Meta
Record Statistics
Record Views
110
Version History
[v1] (Original Submission)
Feb 24, 2023
Verified by curator on
Feb 24, 2023
This Version Number
v1
Citations
Most Recent
This Version
URL Here
https://psecommunity.org/LAPSE:2023.7499
Original Submitter
Auto Uploader for LAPSE
Links to Related Works