LAPSE:2023.6868
Published Article
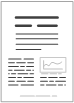
LAPSE:2023.6868
Representation Learning for Detecting the Faults in a Wind Turbine Hydraulic Pitch System Using Deep Learning
February 24, 2023
Wind turbine operators usually use data from a Supervisory Control and Data Acquisition system to monitor their conditions, but it is challenging to make decisions about maintenance based on hundreds of different parameters. Information is often hidden within measurements that operators are unaware of. Therefore, different feature extraction techniques are recommended. The pitch system is of particular importance, and operators are highly motivated to search for effective monitoring solutions. This study investigated different dimensionality reduction techniques for monitoring a hydraulic pitch system in wind turbines. These techniques include principal component analysis (PCA), kernel PCA and a deep autoencoder. Their effectiveness was evaluated based on the performance of a support vector machine classifier whose input space is the new extracted feature set. The developed methodology has been applied to data from a wind farm consisting of five 2.3 MW fixed-speed onshore wind turbines. The available dataset is composed of nine pitch events representing normal and faulty classes. The results indicate that the features extracted by the deep autoencoder are more informative than those extracted by PCA and kernel PCA. These features led to the achievement of a 95.5% F1-score, proving its superiority over the traditional usage of original features.
Record ID
Keywords
deep autoencoder, Fault Detection, feature extraction, pitch system, SCADA, wind turbine
Subject
Suggested Citation
Korkos P, Kleemola J, Linjama M, Lehtovaara A. Representation Learning for Detecting the Faults in a Wind Turbine Hydraulic Pitch System Using Deep Learning. (2023). LAPSE:2023.6868
Author Affiliations
Korkos P: Tribology and Machine Elements, Materials Science and Environmental Engineering, Faculty of Engineering and Natural Sciences, Tampere University, P.O. Box 589, 33014 Tampere, Finland [ORCID]
Kleemola J: Suomen Hyƶtytuuli Oy, P.O. Box 305, 28601 Pori, Finland
Linjama M: Automation Technology and Mechanical Engineering Unit, Faculty of Engineering and Natural Sciences, Tampere University, P.O. Box 589, 33014 Tampere, Finland
Lehtovaara A: Tribology and Machine Elements, Materials Science and Environmental Engineering, Faculty of Engineering and Natural Sciences, Tampere University, P.O. Box 589, 33014 Tampere, Finland
Kleemola J: Suomen Hyƶtytuuli Oy, P.O. Box 305, 28601 Pori, Finland
Linjama M: Automation Technology and Mechanical Engineering Unit, Faculty of Engineering and Natural Sciences, Tampere University, P.O. Box 589, 33014 Tampere, Finland
Lehtovaara A: Tribology and Machine Elements, Materials Science and Environmental Engineering, Faculty of Engineering and Natural Sciences, Tampere University, P.O. Box 589, 33014 Tampere, Finland
Journal Name
Energies
Volume
15
Issue
24
First Page
9279
Year
2022
Publication Date
2022-12-07
Published Version
ISSN
1996-1073
Version Comments
Original Submission
Other Meta
PII: en15249279, Publication Type: Journal Article
Record Map
Published Article
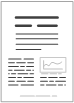
LAPSE:2023.6868
This Record
External Link
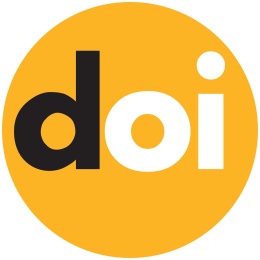
doi:10.3390/en15249279
Publisher Version
Download
Meta
Record Statistics
Record Views
77
Version History
[v1] (Original Submission)
Feb 24, 2023
Verified by curator on
Feb 24, 2023
This Version Number
v1
Citations
Most Recent
This Version
URL Here
https://psecommunity.org/LAPSE:2023.6868
Original Submitter
Auto Uploader for LAPSE
Links to Related Works