LAPSE:2023.36859
Published Article
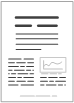
LAPSE:2023.36859
An Improved Fault Diagnosis Approach for Pumps Based on Neural Networks with Improved Adaptive Activation Function
November 30, 2023
Due to the complex underground environment, pumping machines are prone to producing numerous failures. The indicator diagrams of faults are similar to a certain degree, which produces indistinguishable samples. As the samples increase, manual diagnosis becomes difficult, which decreases the accuracy of fault diagnosis. To accurately and quickly judge the fault type, we propose an improved adaptive activation function and apply it to five types of neural networks. The adaptive activation function improves the negative semi-axis slope of the Rectifying linear unit activation function by combining the gated channel conversion unit to improve the performance of the deep learning model. The proposed adaptive activation function is compared to the traditional activation function through the fault diagnosis data set and the public data set. The results show that the activation function has better nonlinearity and can improve the generalization performance of the deep learning model and the accuracy of fault diagnosis. In addition, the proposed adaptive activation function can also be well-embedded into other neural networks.
Record ID
Keywords
adaptive activation function, deep learning, fault diagnosis, pumping unit
Subject
Suggested Citation
Zhang F, Li Y, Shan D, Liu Y, Ma F. An Improved Fault Diagnosis Approach for Pumps Based on Neural Networks with Improved Adaptive Activation Function. (2023). LAPSE:2023.36859
Author Affiliations
Zhang F: School of Information and Automation Engineering, Qilu University of Technology (Shandong Academy of Sciences), Jinan 250300, China [ORCID]
Li Y: School of Information and Automation Engineering, Qilu University of Technology (Shandong Academy of Sciences), Jinan 250300, China
Shan D: School of Mechanical Engineering, Qilu University of Technology (Shandong Academy of Sciences), Jinan 250300, China; System Control and Information Processing Lab, Aerospace Information University, Jinan 250200, China [ORCID]
Liu Y: School of Information and Electrical Engineering, Northeast Petroleum University, Daqing 163319, China
Ma F: School of Information and Automation Engineering, Qilu University of Technology (Shandong Academy of Sciences), Jinan 250300, China
Li Y: School of Information and Automation Engineering, Qilu University of Technology (Shandong Academy of Sciences), Jinan 250300, China
Shan D: School of Mechanical Engineering, Qilu University of Technology (Shandong Academy of Sciences), Jinan 250300, China; System Control and Information Processing Lab, Aerospace Information University, Jinan 250200, China [ORCID]
Liu Y: School of Information and Electrical Engineering, Northeast Petroleum University, Daqing 163319, China
Ma F: School of Information and Automation Engineering, Qilu University of Technology (Shandong Academy of Sciences), Jinan 250300, China
Journal Name
Processes
Volume
11
Issue
9
First Page
2540
Year
2023
Publication Date
2023-08-24
Published Version
ISSN
2227-9717
Version Comments
Original Submission
Other Meta
PII: pr11092540, Publication Type: Journal Article
Record Map
Published Article
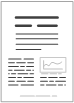
LAPSE:2023.36859
This Record
External Link
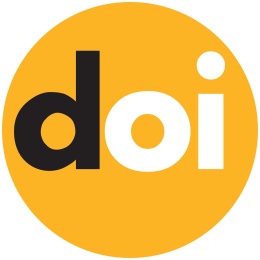
doi:10.3390/pr11092540
Publisher Version
Download
Meta
Record Statistics
Record Views
67
Version History
[v1] (Original Submission)
Nov 30, 2023
Verified by curator on
Nov 30, 2023
This Version Number
v1
Citations
Most Recent
This Version
URL Here
https://psecommunity.org/LAPSE:2023.36859
Original Submitter
Calvin Tsay
Links to Related Works