LAPSE:2023.36792
Published Article
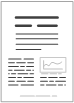
LAPSE:2023.36792
Fused Data-Driven Approach for Early Warning Method of Abnormal Conditions in Chemical Process
September 21, 2023
The utilization of data-driven methods in chemical process modeling has been extensively acknowledged due to their effectiveness. However, with the increasing complexity and variability of chemical processes, predicting and warning of anomalous conditions have become challenging. Extracting valuable features and constructing relevant warning models are critical problems that require resolution. This research proposed a novel fused method that integrates K-means density-based spatial clustering of applications with noise (DBSCAN) clustering and bi-directional long short-term memory multilayer perceptron (Bi-LSTM-MLP) to enable early warning of abnormal conditions in chemical processes. The paper applied the proposed method to analyze the early warning using actual process data from Eastman Tennessee and the atmospheric pressure reduction unit as an example. In the TE model and example, the root mean square error (RMSE) of this method is 0.006855 and 0.052546, respectively, which is quite low when compared to other methods. The experimental results confirmed the effectiveness of our approach.
Record ID
Keywords
abnormal conditions, chemical process, data-driven
Suggested Citation
Song X, Yin F, Zhao D. Fused Data-Driven Approach for Early Warning Method of Abnormal Conditions in Chemical Process. (2023). LAPSE:2023.36792
Author Affiliations
Song X: College of Mechanical and Electrical Engineering, China University of Petroleum (East China), Qingdao 266580, China [ORCID]
Yin F: Center for Safety, Environmental & Engineering, China University of Petroleum (East China), Qingdao 266580, China
Zhao D: College of Chemical Engineering, China University of Petroleum (East China), Qingdao 266580, China [ORCID]
Yin F: Center for Safety, Environmental & Engineering, China University of Petroleum (East China), Qingdao 266580, China
Zhao D: College of Chemical Engineering, China University of Petroleum (East China), Qingdao 266580, China [ORCID]
Journal Name
Processes
Volume
11
Issue
8
First Page
2435
Year
2023
Publication Date
2023-08-12
Published Version
ISSN
2227-9717
Version Comments
Original Submission
Other Meta
PII: pr11082435, Publication Type: Journal Article
Record Map
Published Article
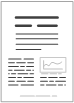
LAPSE:2023.36792
This Record
External Link
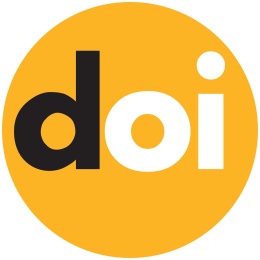
doi:10.3390/pr11082435
Publisher Version
Download
Meta
Record Statistics
Record Views
126
Version History
[v1] (Original Submission)
Sep 21, 2023
Verified by curator on
Sep 21, 2023
This Version Number
v1
Citations
Most Recent
This Version
URL Here
https://psecommunity.org/LAPSE:2023.36792
Original Submitter
Calvin Tsay
Links to Related Works