LAPSE:2023.36625
Published Article
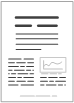
LAPSE:2023.36625
YOLOv7-Based Anomaly Detection Using Intensity and NG Types in Labeling in Cosmetic Manufacturing Processes
September 20, 2023
The advent of the Fourth Industrial Revolution has revolutionized the manufacturing sector by integrating artificial intelligence into vision inspection systems to improve the efficiency and quality of products. Supervised-learning-based vision inspection systems have emerged as a powerful tool for automated quality control in various industries. During visual inspection or final inspection, a human operator physically inspects a product to determine its condition and categorize it based on their know-how. However, the know-how-based visual inspection process is limited in time and space and is affected by many factors. High accuracy in vision inspection is highly dependent on the quality and precision of the labeling process. Therefore, supervised learning methods of 1-STAGE DETECTION, such as You Only Look Once (YOLO), are utilized in automated inspection to improve accuracy. In this paper, we proposed a labeling method that achieves the highest inspection accuracy among labeling methods such as NG intensity and NG intensity when performing anomaly detection using YOLOv7 in the cosmetics manufacturing process.
Record ID
Keywords
anomaly detection, deep learning, object detection, YOLOv7
Subject
Suggested Citation
Beak S, Han YH, Moon Y, Lee J, Jeong J. YOLOv7-Based Anomaly Detection Using Intensity and NG Types in Labeling in Cosmetic Manufacturing Processes. (2023). LAPSE:2023.36625
Author Affiliations
Beak S: Department of Smart Factory Convergence, Sungkyunkwan University, 2066 Seobu-ro, Jangan-gu, Suwon 16419, Gyeonggi-do, Republic of Korea; AI Machine Vision Smart Factory Lab, Dev, 296, Sandan-ro, Danwon-gu, Ansan-si 15433, Gyeonggi-do, Republic of Korea [ORCID]
Han YH: Department of Smart Factory Convergence, Sungkyunkwan University, 2066 Seobu-ro, Jangan-gu, Suwon 16419, Gyeonggi-do, Republic of Korea; AI Machine Vision Smart Factory Lab, Dev, 296, Sandan-ro, Danwon-gu, Ansan-si 15433, Gyeonggi-do, Republic of Korea
Moon Y: Department of Smart Factory Convergence, Sungkyunkwan University, 2066 Seobu-ro, Jangan-gu, Suwon 16419, Gyeonggi-do, Republic of Korea [ORCID]
Lee J: Department of Smart Factory Convergence, Sungkyunkwan University, 2066 Seobu-ro, Jangan-gu, Suwon 16419, Gyeonggi-do, Republic of Korea [ORCID]
Jeong J: Department of Smart Factory Convergence, Sungkyunkwan University, 2066 Seobu-ro, Jangan-gu, Suwon 16419, Gyeonggi-do, Republic of Korea [ORCID]
Han YH: Department of Smart Factory Convergence, Sungkyunkwan University, 2066 Seobu-ro, Jangan-gu, Suwon 16419, Gyeonggi-do, Republic of Korea; AI Machine Vision Smart Factory Lab, Dev, 296, Sandan-ro, Danwon-gu, Ansan-si 15433, Gyeonggi-do, Republic of Korea
Moon Y: Department of Smart Factory Convergence, Sungkyunkwan University, 2066 Seobu-ro, Jangan-gu, Suwon 16419, Gyeonggi-do, Republic of Korea [ORCID]
Lee J: Department of Smart Factory Convergence, Sungkyunkwan University, 2066 Seobu-ro, Jangan-gu, Suwon 16419, Gyeonggi-do, Republic of Korea [ORCID]
Jeong J: Department of Smart Factory Convergence, Sungkyunkwan University, 2066 Seobu-ro, Jangan-gu, Suwon 16419, Gyeonggi-do, Republic of Korea [ORCID]
Journal Name
Processes
Volume
11
Issue
8
First Page
2266
Year
2023
Publication Date
2023-07-27
Published Version
ISSN
2227-9717
Version Comments
Original Submission
Other Meta
PII: pr11082266, Publication Type: Journal Article
Record Map
Published Article
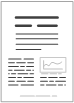
LAPSE:2023.36625
This Record
External Link
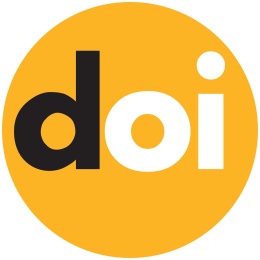
doi:10.3390/pr11082266
Publisher Version
Download
Meta
Record Statistics
Record Views
100
Version History
[v1] (Original Submission)
Sep 20, 2023
Verified by curator on
Sep 20, 2023
This Version Number
v1
Citations
Most Recent
This Version
URL Here
https://psecommunity.org/LAPSE:2023.36625
Original Submitter
Calvin Tsay
Links to Related Works