LAPSE:2023.36481
Published Article
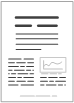
LAPSE:2023.36481
Dimension Reduction and Classifier-Based Feature Selection for Oversampled Gene Expression Data and Cancer Classification
August 2, 2023
Gene expression data are usually known for having a large number of features. Usually, some of these features are irrelevant and redundant. However, in some cases, all features, despite being numerous, show high importance and contribute to the data analysis. In a similar fashion, gene expression data sometimes have limited instances with a high rate of imbalance among the classes. This can limit the exposure of a classification model to instances of different categories, thereby influencing the performance of the model. In this study, we proposed a cancer detection approach that utilized data preprocessing techniques such as oversampling, feature selection, and classification models. The study used SVMSMOTE for the oversampling of the six examined datasets. Further, we examined different techniques for feature selection using dimension reduction methods and classifier-based feature ranking and selection. We trained six machine learning algorithms, using repeated 5-fold cross-validation on different microarray datasets. The performance of the algorithms differed based on the data and feature reduction technique used.
Record ID
Keywords
cancer classification, gene expression, Machine Learning, microarray data, sampling methods
Subject
Suggested Citation
Petinrin OO, Saeed F, Salim N, Toseef M, Liu Z, Muyide IO. Dimension Reduction and Classifier-Based Feature Selection for Oversampled Gene Expression Data and Cancer Classification. (2023). LAPSE:2023.36481
Author Affiliations
Petinrin OO: Department of Computer Science, City University of Hong Kong, Kowloon, Hong Kong [ORCID]
Saeed F: DAAI Research Group, Department of Computing and Data Science, School of Computing and Digital Technology, Birmingham City University, Birmingham B4 7XG, UK [ORCID]
Salim N: UTM Big Data Centre, Ibnu Sina Institute for Scientific and Industrial Research, Universiti Teknologi Malaysia, Johor Bahru 81310, Johor, Malaysia
Toseef M: Department of Computer Science, City University of Hong Kong, Kowloon, Hong Kong
Liu Z: Department of Computer Science, City University of Hong Kong, Kowloon, Hong Kong [ORCID]
Muyide IO: College of Computing, Georgia Institute of Technology, Atlanta, GA 30332, USA [ORCID]
Saeed F: DAAI Research Group, Department of Computing and Data Science, School of Computing and Digital Technology, Birmingham City University, Birmingham B4 7XG, UK [ORCID]
Salim N: UTM Big Data Centre, Ibnu Sina Institute for Scientific and Industrial Research, Universiti Teknologi Malaysia, Johor Bahru 81310, Johor, Malaysia
Toseef M: Department of Computer Science, City University of Hong Kong, Kowloon, Hong Kong
Liu Z: Department of Computer Science, City University of Hong Kong, Kowloon, Hong Kong [ORCID]
Muyide IO: College of Computing, Georgia Institute of Technology, Atlanta, GA 30332, USA [ORCID]
Journal Name
Processes
Volume
11
Issue
7
First Page
1940
Year
2023
Publication Date
2023-06-27
Published Version
ISSN
2227-9717
Version Comments
Original Submission
Other Meta
PII: pr11071940, Publication Type: Journal Article
Record Map
Published Article
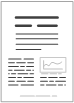
LAPSE:2023.36481
This Record
External Link
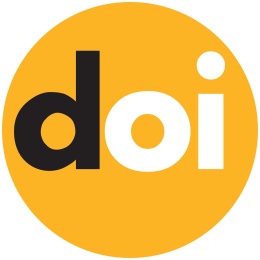
doi:10.3390/pr11071940
Publisher Version
Download
Meta
Record Statistics
Record Views
107
Version History
[v1] (Original Submission)
Aug 2, 2023
Verified by curator on
Aug 2, 2023
This Version Number
v1
Citations
Most Recent
This Version
URL Here
https://psecommunity.org/LAPSE:2023.36481
Original Submitter
Calvin Tsay
Links to Related Works