LAPSE:2023.36328
Published Article
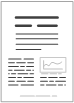
LAPSE:2023.36328
Synthetic Minority Oversampling Enhanced FEM for Tool Wear Condition Monitoring
July 7, 2023
Recent advances in artificial intelligence (AI) technology have led to increasing interest in the development of AI-based tool wear condition monitoring methods, heavily relying on large training samples. However, the high cost of tool wear experiment and the uncertainty of tool wear change in the machining process lead to the problems of sample missing and insufficiency in the model training stage, which seriously affects the identification accuracy of many AI models. In this paper, a novel identification method based on finite-element modeling (FEM) and the synthetic minority oversampling technique (SMOTE) is proposed to overcome the problem of sample missing and sample insufficiency. Firstly, a few tool wear monitoring experiments are carried out to obtain experimental samples with low cost. Then, a FEM model based on the Johnson−Cook constitutive model was established and verified according to the experimental samples. Based on the verified FEM model, the simulated missing sample in the experiments can be supplemented to compose a complete training set. Finally, the SMOTE is employed to expand the sample size to construct a perfect training set to train the SVM classification model. End milling tool wear monitoring experiments demonstrate that the proposed FEM-SMOTE method can obtain 98.7% identification accuracy, which is 30% higher than that based on experimental samples. The proposed method provides an effective approach for tool wear condition monitoring with low experimental cost.
Record ID
Keywords
finite-element modeling, sample missing and insufficiency, synthetic minority oversampling technique, tool wear condition
Subject
Suggested Citation
Zhou Y, Ye C, Huang D, Peng B, Sun B, Zhang H. Synthetic Minority Oversampling Enhanced FEM for Tool Wear Condition Monitoring. (2023). LAPSE:2023.36328
Author Affiliations
Zhou Y: School of Information Science and Technology, Northwest University, Xi’an 710127, China; College of Mechanical and Electrical Engineering, Wenzhou University, Wenzhou 325035, China [ORCID]
Ye C: Zhejiang Meishuo Electric Technology Co., Ltd., Wenzhou 325699, China
Huang D: Zhejiang Meishuo Electric Technology Co., Ltd., Wenzhou 325699, China
Peng B: Zhejiang Meishuo Electric Technology Co., Ltd., Wenzhou 325699, China
Sun B: College of Mechanical and Electrical Engineering, Wenzhou University, Wenzhou 325035, China
Zhang H: Laser and Optoelectronic Intelligent Manufacturing Research Institute, Wenzhou University, Wenzhou 325025, China
Ye C: Zhejiang Meishuo Electric Technology Co., Ltd., Wenzhou 325699, China
Huang D: Zhejiang Meishuo Electric Technology Co., Ltd., Wenzhou 325699, China
Peng B: Zhejiang Meishuo Electric Technology Co., Ltd., Wenzhou 325699, China
Sun B: College of Mechanical and Electrical Engineering, Wenzhou University, Wenzhou 325035, China
Zhang H: Laser and Optoelectronic Intelligent Manufacturing Research Institute, Wenzhou University, Wenzhou 325025, China
Journal Name
Processes
Volume
11
Issue
6
First Page
1785
Year
2023
Publication Date
2023-06-12
Published Version
ISSN
2227-9717
Version Comments
Original Submission
Other Meta
PII: pr11061785, Publication Type: Journal Article
Record Map
Published Article
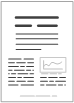
LAPSE:2023.36328
This Record
External Link
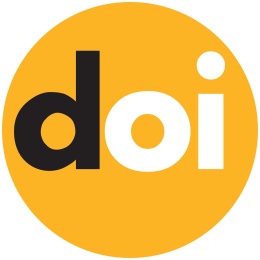
doi:10.3390/pr11061785
Publisher Version
Download
Meta
Record Statistics
Record Views
91
Version History
[v1] (Original Submission)
Jul 7, 2023
Verified by curator on
Jul 7, 2023
This Version Number
v1
Citations
Most Recent
This Version
URL Here
https://psecommunity.org/LAPSE:2023.36328
Original Submitter
Calvin Tsay
Links to Related Works