LAPSE:2023.35928
Published Article
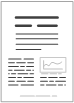
LAPSE:2023.35928
Integration of Multiple Bayesian Optimized Machine Learning Techniques and Conventional Well Logs for Accurate Prediction of Porosity in Carbonate Reservoirs
June 7, 2023
The accurate estimation of reservoir porosity plays a vital role in estimating the amount of hydrocarbon reserves and evaluating the economic potential of a reservoir. It also aids decision making during the exploration and development phases of oil and gas fields. This study evaluates the integration of artificial intelligence techniques, conventional well logs, and core analysis for the accurate prediction of porosity in carbonate reservoirs. In general, carbonate reservoirs are characterized by their complex pore systems, with the wide spatial variation and highly nonlinear nature of their petrophysical properties. Therefore, they require detailed well-log interpretations to accurately estimate their properties, making them good candidates for the application of machine learning techniques. Accordingly, a large database of (2100) well-log records and core-porosity measurements were integrated with four state-of-the-art machine learning techniques (multilayer perceptron artificial neural network, MLP-ANN; Gaussian process regression, GPR; least squares gradient boosting ensemble, LS-Boost; and radial basis function neural network, RBF-NN) for the prediction of reservoir porosity. The well-log data used in this study include sonic acoustic travel time, Gamma-ray, and bulk density log records, which were carefully collected from five wells in a carbonate reservoir. This study revealed that all the artificial intelligence models achieved high accuracy, with R-squared values exceeding 90% during both the training and blind-testing phases. Among the AI models examined, the GPR model outperformed the others in terms of the R-squared values, root-mean-square error (RMSE), and coefficient of variation of the root-mean-square error (CVRMSE). Furthermore, this study introduces an artificially intelligent AI-based correlation for the estimation of reservoir porosity from well-log data; this correlation was developed using an in-house, Fortran-coded MLP-ANN model presented herein. This AI-based correlation gave a promising level of accuracy, with R-squared values of 92% and 90% for the training and blind-testing datasets, respectively. This correlation can serve as an accurate and easy-to-use tool for porosity prediction without any prior experience in utilizing or implementing machine learning models.
Record ID
Keywords
carbonate reservoirs, Gaussian process regression, least squares gradient boosting ensemble, machine learning techniques, porosity AI-based correlation
Subject
Suggested Citation
Alatefi S, Abdel Azim R, Alkouh A, Hamada G. Integration of Multiple Bayesian Optimized Machine Learning Techniques and Conventional Well Logs for Accurate Prediction of Porosity in Carbonate Reservoirs. (2023). LAPSE:2023.35928
Author Affiliations
Alatefi S: Department of Petroleum Engineering Technology, College of Technological Studies, PAAET, P.O. Box 42325, Kuwait City 70654, Kuwait [ORCID]
Abdel Azim R: Petroleum Engineering Department, American University of Kurdistan, Erbil 42003, Iraq [ORCID]
Alkouh A: Department of Petroleum Engineering Technology, College of Technological Studies, PAAET, P.O. Box 42325, Kuwait City 70654, Kuwait
Hamada G: Petroleum Engineering Department, American University of Kurdistan, Erbil 42003, Iraq
Abdel Azim R: Petroleum Engineering Department, American University of Kurdistan, Erbil 42003, Iraq [ORCID]
Alkouh A: Department of Petroleum Engineering Technology, College of Technological Studies, PAAET, P.O. Box 42325, Kuwait City 70654, Kuwait
Hamada G: Petroleum Engineering Department, American University of Kurdistan, Erbil 42003, Iraq
Journal Name
Processes
Volume
11
Issue
5
First Page
1339
Year
2023
Publication Date
2023-04-26
Published Version
ISSN
2227-9717
Version Comments
Original Submission
Other Meta
PII: pr11051339, Publication Type: Journal Article
Record Map
Published Article
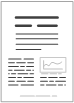
LAPSE:2023.35928
This Record
External Link
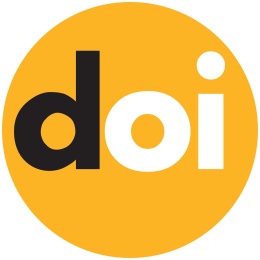
doi:10.3390/pr11051339
Publisher Version
Download
Meta
Record Statistics
Record Views
88
Version History
[v1] (Original Submission)
Jun 7, 2023
Verified by curator on
Jun 7, 2023
This Version Number
v1
Citations
Most Recent
This Version
URL Here
https://psecommunity.org/LAPSE:2023.35928
Original Submitter
Calvin Tsay
Links to Related Works