LAPSE:2023.35793
Published Article
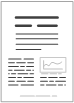
LAPSE:2023.35793
Cloud-Based Deep Learning for Co-Estimation of Battery State of Charge and State of Health
May 23, 2023
Rechargeable lithium-ion batteries are currently the most viable option for energy storage systems in electric vehicle (EV) applications due to their high specific energy, falling costs, and acceptable cycle life. However, accurately predicting the parameters of complex, nonlinear battery systems remains challenging, given diverse aging mechanisms, cell-to-cell variations, and dynamic operating conditions. The states and parameters of batteries are becoming increasingly important in ubiquitous application scenarios, yet our ability to predict cell performance under realistic conditions remains limited. To address the challenge of modelling and predicting the evolution of multiphysics and multiscale battery systems, this study proposes a cloud-based AI-enhanced framework. The framework aims to achieve practical success in the co-estimation of the state of charge (SOC) and state of health (SOH) during the system’s operational lifetime. Self-supervised transformer neural networks offer new opportunities to learn representations of observational data with multiple levels of abstraction and attention mechanisms. Coupling the cloud-edge computing framework with the versatility of deep learning can leverage the predictive ability of exploiting long-range spatio-temporal dependencies across multiple scales.
Record ID
Keywords
cloud, deep learning, field application, lithium-ion battery, state of charge, state of health
Subject
Suggested Citation
Shi D, Zhao J, Wang Z, Zhao H, Eze C, Wang J, Lian Y, Burke AF. Cloud-Based Deep Learning for Co-Estimation of Battery State of Charge and State of Health. (2023). LAPSE:2023.35793
Author Affiliations
Shi D: Hubei Longzhong Laboratory, Hubei University of Arts and Science, Xiangyang 441053, China
Zhao J: Institute of Transportation Studies, University of California-Davis, Davis, CA 95616, USA [ORCID]
Wang Z: Hubei Longzhong Laboratory, Hubei University of Arts and Science, Xiangyang 441053, China
Zhao H: College of Big Data and Internet, Shenzhen Technology University, Shenzhen 518118, China
Eze C: Department of Mechanical Engineering, University of California, Merced, CA 94720, USA [ORCID]
Wang J: BYD Automotive Engineering Research Institute, Shenzhen 518118, China
Lian Y: BYD Automotive Engineering Research Institute, Shenzhen 518118, China
Burke AF: Institute of Transportation Studies, University of California-Davis, Davis, CA 95616, USA
Zhao J: Institute of Transportation Studies, University of California-Davis, Davis, CA 95616, USA [ORCID]
Wang Z: Hubei Longzhong Laboratory, Hubei University of Arts and Science, Xiangyang 441053, China
Zhao H: College of Big Data and Internet, Shenzhen Technology University, Shenzhen 518118, China
Eze C: Department of Mechanical Engineering, University of California, Merced, CA 94720, USA [ORCID]
Wang J: BYD Automotive Engineering Research Institute, Shenzhen 518118, China
Lian Y: BYD Automotive Engineering Research Institute, Shenzhen 518118, China
Burke AF: Institute of Transportation Studies, University of California-Davis, Davis, CA 95616, USA
Journal Name
Energies
Volume
16
Issue
9
First Page
3855
Year
2023
Publication Date
2023-04-30
Published Version
ISSN
1996-1073
Version Comments
Original Submission
Other Meta
PII: en16093855, Publication Type: Journal Article
Record Map
Published Article
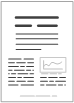
LAPSE:2023.35793
This Record
External Link
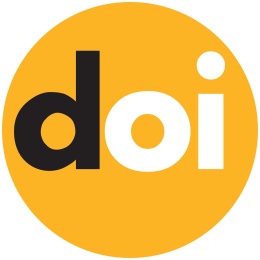
doi:10.3390/en16093855
Publisher Version
Download
Meta
Record Statistics
Record Views
108
Version History
[v1] (Original Submission)
May 23, 2023
Verified by curator on
May 23, 2023
This Version Number
v1
Citations
Most Recent
This Version
URL Here
https://psecommunity.org/LAPSE:2023.35793
Original Submitter
Calvin Tsay
Links to Related Works