LAPSE:2023.35331
Published Article
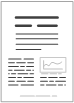
LAPSE:2023.35331
An Energy-Efficiency Prediction Method in Crude Distillation Process Based on Long Short-Term Memory Network
April 28, 2023
The petrochemical industry is a pillar industry for the development of the national economy affecting people’s daily living standards. Crude distillation process is the core and leading unit of the petrochemical industry. Its energy consumption accounts for more than 20% of the total energy consumption of the whole plant, which is the highest energy consumption link. A model based on the long short-term memory network (LSTM) is proposed in this paper to predict and analyze energy efficiency. This model extracts the complex relationship between many process variables and predicts the energy efficiency of the crude distillation process. Firstly, the process simulation of crude distillation is carried out. By adding random disturbance, the data set in different working conditions is obtained, and the difference between the working conditions is expressed with the distance-coded heat map. Secondly, the Savitzky−Golay (SG) filter is used to smooth the data, which preserves the original characteristics of the data and improves the prediction effect. Finally, the LSTM model is used to predict and analyze the energy efficiency of products under different working conditions. The MAE, MSE, and MAPE results of the LSTM model under different working conditions in the test set are lower than 1.3872%, 0.0307%, and 0.2555%, respectively. Therefore, the LSTM model can be considered a perfect model for the test set, and the prediction results have high reliability to accurately predict the energy efficiency of the crude distillation process.
Record ID
Keywords
crude distillation process, energy consumption prediction, Energy Efficiency, long short-term memory
Subject
Suggested Citation
Zhang Y, Cui Z, Wang M, Liu B, Fan X, Tian W. An Energy-Efficiency Prediction Method in Crude Distillation Process Based on Long Short-Term Memory Network. (2023). LAPSE:2023.35331
Author Affiliations
Zhang Y: College of Chemical Engineering, Qingdao University of Science & Technology, Qingdao 266042, China
Cui Z: College of Chemical Engineering, Qingdao University of Science & Technology, Qingdao 266042, China
Wang M: Sinopec Qingdao Petrochemical Co., Ltd., Qingdao 266043, China
Liu B: College of Chemical Engineering, Qingdao University of Science & Technology, Qingdao 266042, China [ORCID]
Fan X: College of Chemical Engineering, Qingdao University of Science & Technology, Qingdao 266042, China
Tian W: College of Chemical Engineering, Qingdao University of Science & Technology, Qingdao 266042, China [ORCID]
Cui Z: College of Chemical Engineering, Qingdao University of Science & Technology, Qingdao 266042, China
Wang M: Sinopec Qingdao Petrochemical Co., Ltd., Qingdao 266043, China
Liu B: College of Chemical Engineering, Qingdao University of Science & Technology, Qingdao 266042, China [ORCID]
Fan X: College of Chemical Engineering, Qingdao University of Science & Technology, Qingdao 266042, China
Tian W: College of Chemical Engineering, Qingdao University of Science & Technology, Qingdao 266042, China [ORCID]
Journal Name
Processes
Volume
11
Issue
4
First Page
1257
Year
2023
Publication Date
2023-04-19
Published Version
ISSN
2227-9717
Version Comments
Original Submission
Other Meta
PII: pr11041257, Publication Type: Journal Article
Record Map
Published Article
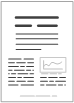
LAPSE:2023.35331
This Record
External Link
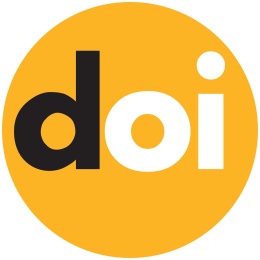
doi:10.3390/pr11041257
Publisher Version
Download
Meta
Record Statistics
Record Views
121
Version History
[v1] (Original Submission)
Apr 28, 2023
Verified by curator on
Apr 28, 2023
This Version Number
v1
Citations
Most Recent
This Version
URL Here
https://psecommunity.org/LAPSE:2023.35331
Original Submitter
Auto Uploader for LAPSE
Links to Related Works