LAPSE:2023.35228
Published Article
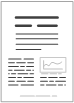
LAPSE:2023.35228
A Novel Prediction Method Based on Bi-Channel Hierarchical Vision Transformer for Rolling Bearings’ Remaining Useful Life
April 28, 2023
Accurate prediction of the remaining useful life (RUL) of rolling bearings can effectively ensure the safety of complicated machinery and equipment in service. However, the diversity of rolling bearing degradation processes makes it difficult for deep learning-based RUL prediction methods to improve prediction accuracy further and provide generalizability for engineering applications. This study proposed a novelty RUL prediction model for rolling bearings based on a bi-channel hierarchical vision transformer to reduce the impact of the above problems on prediction accuracy improvement. Firstly, hierarchical vision transformer network structures based on different-sized patches were employed to extract depth features containing more degradation processes information from input samples. Second, the dual channel fusion method is implemented into classic RUL prediction networks based on a multi-layer fully connected network to improve prediction accuracy. With two distinct validation experimental arrangements utilizing the datasets from PHM 2012, the prediction accuracy of the proposed approach can be increased by up to 9.43% and 43.10%, respectively, compared with the current standard method. The results demonstrate that the proposed method is more suitable for rolling bearing RUL prediction.
Record ID
Keywords
channel fusion, deep learning, hierarchical vision transformer, remaining useful life prediction, rolling bearing
Subject
Suggested Citation
Hao W, Li Z, Qin G, Ding K, Lai X, Zhang K. A Novel Prediction Method Based on Bi-Channel Hierarchical Vision Transformer for Rolling Bearings’ Remaining Useful Life. (2023). LAPSE:2023.35228
Author Affiliations
Hao W: Department of Information Technology, CRRC Qingdao Sifang Limited Company, Qingdao 266111, China
Li Z: School of Mechanical Engineering, Southwest Jiaotong University, Chengdu 610031, China [ORCID]
Qin G: School of Mechanical Engineering, Southwest Jiaotong University, Chengdu 610031, China
Ding K: School of Mechanical Engineering, Southwest Jiaotong University, Chengdu 610031, China
Lai X: School of Mechanical Engineering, Southwest Jiaotong University, Chengdu 610031, China
Zhang K: School of Mechanical Engineering, Southwest Jiaotong University, Chengdu 610031, China
Li Z: School of Mechanical Engineering, Southwest Jiaotong University, Chengdu 610031, China [ORCID]
Qin G: School of Mechanical Engineering, Southwest Jiaotong University, Chengdu 610031, China
Ding K: School of Mechanical Engineering, Southwest Jiaotong University, Chengdu 610031, China
Lai X: School of Mechanical Engineering, Southwest Jiaotong University, Chengdu 610031, China
Zhang K: School of Mechanical Engineering, Southwest Jiaotong University, Chengdu 610031, China
Journal Name
Processes
Volume
11
Issue
4
First Page
1153
Year
2023
Publication Date
2023-04-09
Published Version
ISSN
2227-9717
Version Comments
Original Submission
Other Meta
PII: pr11041153, Publication Type: Journal Article
Record Map
Published Article
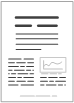
LAPSE:2023.35228
This Record
External Link
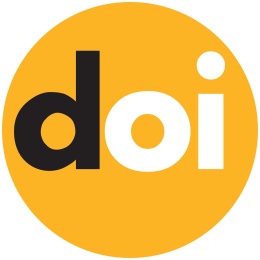
doi:10.3390/pr11041153
Publisher Version
Download
Meta
Record Statistics
Record Views
90
Version History
[v1] (Original Submission)
Apr 28, 2023
Verified by curator on
Apr 28, 2023
This Version Number
v1
Citations
Most Recent
This Version
URL Here
https://psecommunity.org/LAPSE:2023.35228
Original Submitter
Auto Uploader for LAPSE
Links to Related Works