LAPSE:2023.35081
Published Article
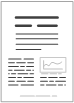
LAPSE:2023.35081
Machine Learning Methods in Skin Disease Recognition: A Systematic Review
April 28, 2023
Skin lesions affect millions of people worldwide. They can be easily recognized based on their typically abnormal texture and color but are difficult to diagnose due to similar symptoms among certain types of lesions. The motivation for this study is to collate and analyze machine learning (ML) applications in skin lesion research, with the goal of encouraging the development of automated systems for skin disease diagnosis. To assist dermatologists in their clinical diagnosis, several skin image datasets have been developed and published online. Such efforts have motivated researchers and medical staff to develop automatic skin diagnosis systems using image segmentation and classification processes. This paper summarizes the fundamental steps in skin lesion diagnosis based on papers mainly published since 2013. The applications of ML methods (including traditional ML and deep learning (DL)) in skin disease recognition are reviewed based on their contributions, methods, and achieved results. Such technical analysis is beneficial to the continuing development of reliable and effective computer-aided skin disease diagnosis systems. We believe that more research efforts will lead to the current automatic skin diagnosis studies being used in real clinical settings in the near future.
Record ID
Keywords
computer assisted diagnostics, deep learning, dermatology, Machine Learning, skin image segmentation, skin lesion classification
Subject
Suggested Citation
Sun J, Yao K, Huang G, Zhang C, Leach M, Huang K, Yang X. Machine Learning Methods in Skin Disease Recognition: A Systematic Review. (2023). LAPSE:2023.35081
Author Affiliations
Sun J: School of Advanced Technology, Xi’an Jiaotong-Liverpool University, Suzhou 215123, China [ORCID]
Yao K: School of Advanced Technology, Xi’an Jiaotong-Liverpool University, Suzhou 215123, China; School of Engineering, University of Liverpool, Liverpool L69 3BX, UK [ORCID]
Huang G: School of Advanced Technology, Xi’an Jiaotong-Liverpool University, Suzhou 215123, China
Zhang C: School of Advanced Technology, Xi’an Jiaotong-Liverpool University, Suzhou 215123, China; School of Engineering, University of Liverpool, Liverpool L69 3BX, UK [ORCID]
Leach M: School of Advanced Technology, Xi’an Jiaotong-Liverpool University, Suzhou 215123, China [ORCID]
Huang K: Data Science Research Centre, Duke Kunshan University, Kunshan 215316, China [ORCID]
Yang X: School of Advanced Technology, Xi’an Jiaotong-Liverpool University, Suzhou 215123, China
Yao K: School of Advanced Technology, Xi’an Jiaotong-Liverpool University, Suzhou 215123, China; School of Engineering, University of Liverpool, Liverpool L69 3BX, UK [ORCID]
Huang G: School of Advanced Technology, Xi’an Jiaotong-Liverpool University, Suzhou 215123, China
Zhang C: School of Advanced Technology, Xi’an Jiaotong-Liverpool University, Suzhou 215123, China; School of Engineering, University of Liverpool, Liverpool L69 3BX, UK [ORCID]
Leach M: School of Advanced Technology, Xi’an Jiaotong-Liverpool University, Suzhou 215123, China [ORCID]
Huang K: Data Science Research Centre, Duke Kunshan University, Kunshan 215316, China [ORCID]
Yang X: School of Advanced Technology, Xi’an Jiaotong-Liverpool University, Suzhou 215123, China
Journal Name
Processes
Volume
11
Issue
4
First Page
1003
Year
2023
Publication Date
2023-03-26
Published Version
ISSN
2227-9717
Version Comments
Original Submission
Other Meta
PII: pr11041003, Publication Type: Review
Record Map
Published Article
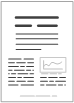
LAPSE:2023.35081
This Record
External Link
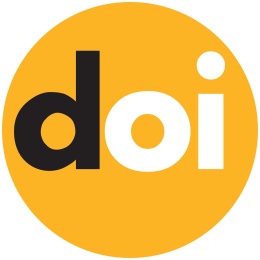
doi:10.3390/pr11041003
Publisher Version
Download
Meta
Record Statistics
Record Views
105
Version History
[v1] (Original Submission)
Apr 28, 2023
Verified by curator on
Apr 28, 2023
This Version Number
v1
Citations
Most Recent
This Version
URL Here
https://psecommunity.org/LAPSE:2023.35081
Original Submitter
Auto Uploader for LAPSE
Links to Related Works