LAPSE:2023.34776
Published Article
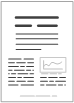
LAPSE:2023.34776
AI-Based Scheduling Models, Optimization, and Prediction for Hydropower Generation: Opportunities, Issues, and Future Directions
April 28, 2023
Hydropower is the most prevalent source of renewable energy production worldwide. As the global demand for robust and ecologically sustainable energy production increases, developing and enhancing the current energy production processes is essential. In the past decade, machine learning has contributed significantly to various fields, and hydropower is no exception. All three horizons of hydropower models could benefit from machine learning: short-term, medium-term, and long-term. Currently, dynamic programming is used in the majority of hydropower scheduling models. In this paper, we review the present state of the hydropower scheduling problem as well as the development of machine learning as a type of optimization problem and prediction tool. To the best of our knowledge, this is the first survey article that provides a comprehensive overview of machine learning and artificial intelligence applications in the hydroelectric power industry for scheduling, optimization, and prediction.
Record ID
Keywords
deep neural networks, hydropower, hydropower scheduling, linear regression, Machine Learning, Optimization, random forest, reinforcement learning, stochastic programming
Subject
Suggested Citation
Villeneuve Y, Séguin S, Chehri A. AI-Based Scheduling Models, Optimization, and Prediction for Hydropower Generation: Opportunities, Issues, and Future Directions. (2023). LAPSE:2023.34776
Author Affiliations
Villeneuve Y: Group for Research in Decision Analysis (GERAD), Université du Québec à Chicoutimi, Chicoutimi, QC G7H 2B1, Canada [ORCID]
Séguin S: Group for Research in Decision Analysis (GERAD), Université du Québec à Chicoutimi, Chicoutimi, QC G7H 2B1, Canada
Chehri A: Department of Mathematics and Computer Science, Royal Military College of Canada, Kingston, ON K7K 7B4, Canada [ORCID]
Séguin S: Group for Research in Decision Analysis (GERAD), Université du Québec à Chicoutimi, Chicoutimi, QC G7H 2B1, Canada
Chehri A: Department of Mathematics and Computer Science, Royal Military College of Canada, Kingston, ON K7K 7B4, Canada [ORCID]
Journal Name
Energies
Volume
16
Issue
8
First Page
3335
Year
2023
Publication Date
2023-04-09
Published Version
ISSN
1996-1073
Version Comments
Original Submission
Other Meta
PII: en16083335, Publication Type: Review
Record Map
Published Article
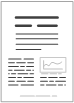
LAPSE:2023.34776
This Record
External Link
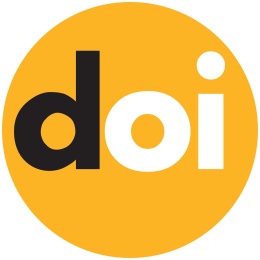
doi:10.3390/en16083335
Publisher Version
Download
Meta
Record Statistics
Record Views
106
Version History
[v1] (Original Submission)
Apr 28, 2023
Verified by curator on
Apr 28, 2023
This Version Number
v1
Citations
Most Recent
This Version
URL Here
https://psecommunity.org/LAPSE:2023.34776
Original Submitter
Auto Uploader for LAPSE
Links to Related Works