LAPSE:2023.34216
Published Article
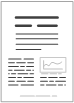
LAPSE:2023.34216
A Novel Accurate and Fast Converging Deep Learning-Based Model for Electrical Energy Consumption Forecasting in a Smart Grid
April 25, 2023
Energy consumption forecasting is of prime importance for the restructured environment of energy management in the electricity market. Accurate energy consumption forecasting is essential for efficient energy management in the smart grid (SG); however, the energy consumption pattern is non-linear with a high level of uncertainty and volatility. Forecasting such complex patterns requires accurate and fast forecasting models. In this paper, a novel hybrid electrical energy consumption forecasting model is proposed based on a deep learning model known as factored conditional restricted Boltzmann machine (FCRBM). The deep learning-based FCRBM model uses a rectified linear unit (ReLU) activation function and a multivariate autoregressive technique for the network training. The proposed model predicts future electrical energy consumption for efficient energy management in the SG. The proposed model is a novel hybrid model comprising four modules: (i) data processing and features selection module, (ii) deep learning-based FCRBM forecasting module, (iii) genetic wind driven optimization (GWDO) algorithm-based optimization module, and (iv) utilization module. The proposed hybrid model, called FS-FCRBM-GWDO, is tested and evaluated on real power grid data of USA in terms of four performance metrics: mean absolute percentage deviation (MAPD), variance, correlation coefficient, and convergence rate. Simulation results validate that the proposed hybrid FS-FCRBM-GWDO model has superior performance than existing models such as accurate fast converging short-term load forecasting (AFC-STLF) model, mutual information-modified enhanced differential evolution algorithm-artificial neural network (MI-mEDE-ANN)-based model, features selection-ANN (FS-ANN)-based model, and Bi-level model, in terms of forecast accuracy and convergence rate.
Record ID
Keywords
deep learning, electric energy consumption forecasting, factored conditional restricted Boltzmann machine, heuristic optimization algorithm, modified feature selection technique, rectified linear unit, smart grid
Subject
Suggested Citation
Hafeez G, Alimgeer KS, Wadud Z, Shafiq Z, Ali Khan MU, Khan I, Khan FA, Derhab A. A Novel Accurate and Fast Converging Deep Learning-Based Model for Electrical Energy Consumption Forecasting in a Smart Grid. (2023). LAPSE:2023.34216
Author Affiliations
Hafeez G: Department of Electrical and Computer Engineering, COMSATS University Islamabad, Islamabad Campus 44000, Pakistan; Department of Electrical Engineering, University of Engineering and Technology, Mardan 23200, Pakistan [ORCID]
Alimgeer KS: Department of Electrical and Computer Engineering, COMSATS University Islamabad, Islamabad Campus 44000, Pakistan [ORCID]
Wadud Z: Department of Computer Systems Engineering, University of Engineering and Technology Peshawar, Peshawar 25000, Pakistan
Shafiq Z: Department of Electrical Engineering, University of Engineering and Technology, Mardan 23200, Pakistan
Ali Khan MU: Department of Electrical Engineering, University of Engineering and Technology Peshawar, Peshawar 25000, Pakistan
Khan I: Department of Electrical Engineering, University of Engineering and Technology, Mardan 23200, Pakistan [ORCID]
Khan FA: Center of Excellence in Information Assurance, King Saud University, Riyadh 11653, Saudi Arabia [ORCID]
Derhab A: Center of Excellence in Information Assurance, King Saud University, Riyadh 11653, Saudi Arabia [ORCID]
Alimgeer KS: Department of Electrical and Computer Engineering, COMSATS University Islamabad, Islamabad Campus 44000, Pakistan [ORCID]
Wadud Z: Department of Computer Systems Engineering, University of Engineering and Technology Peshawar, Peshawar 25000, Pakistan
Shafiq Z: Department of Electrical Engineering, University of Engineering and Technology, Mardan 23200, Pakistan
Ali Khan MU: Department of Electrical Engineering, University of Engineering and Technology Peshawar, Peshawar 25000, Pakistan
Khan I: Department of Electrical Engineering, University of Engineering and Technology, Mardan 23200, Pakistan [ORCID]
Khan FA: Center of Excellence in Information Assurance, King Saud University, Riyadh 11653, Saudi Arabia [ORCID]
Derhab A: Center of Excellence in Information Assurance, King Saud University, Riyadh 11653, Saudi Arabia [ORCID]
Journal Name
Energies
Volume
13
Issue
9
Article Number
E2244
Year
2020
Publication Date
2020-05-03
Published Version
ISSN
1996-1073
Version Comments
Original Submission
Other Meta
PII: en13092244, Publication Type: Journal Article
Record Map
Published Article
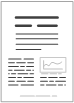
LAPSE:2023.34216
This Record
External Link
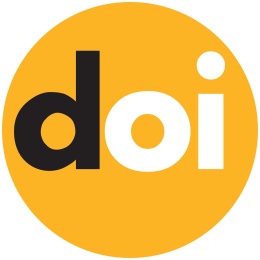
doi:10.3390/en13092244
Publisher Version
Download
Meta
Record Statistics
Record Views
81
Version History
[v1] (Original Submission)
Apr 25, 2023
Verified by curator on
Apr 25, 2023
This Version Number
v1
Citations
Most Recent
This Version
URL Here
https://psecommunity.org/LAPSE:2023.34216
Original Submitter
Auto Uploader for LAPSE
Links to Related Works