LAPSE:2023.34127
Published Article
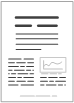
LAPSE:2023.34127
A Machine Learning-Based Gradient Boosting Regression Approach for Wind Power Production Forecasting: A Step towards Smart Grid Environments
April 25, 2023
In the last few years, several countries have accomplished their determined renewable energy targets to achieve their future energy requirements with the foremost aim to encourage sustainable growth with reduced emissions, mainly through the implementation of wind and solar energy. In the present study, we propose and compare five optimized robust regression machine learning methods, namely, random forest, gradient boosting machine (GBM), k-nearest neighbor (kNN), decision-tree, and extra tree regression, which are applied to improve the forecasting accuracy of short-term wind energy generation in the Turkish wind farms, situated in the west of Turkey, on the basis of a historic data of the wind speed and direction. Polar diagrams are plotted and the impacts of input variables such as the wind speed and direction on the wind energy generation are examined. Scatter curves depicting relationships between the wind speed and the produced turbine power are plotted for all of the methods and the predicted average wind power is compared with the real average power from the turbine with the help of the plotted error curves. The results demonstrate the superior forecasting performance of the algorithm incorporating gradient boosting machine regression.
Record ID
Keywords
Machine Learning, Renewable and Sustainable Energy, Renewable and Sustainable Energy, smart grid environment, wind power generation
Subject
Suggested Citation
Singh U, Rizwan M, Alaraj M, Alsaidan I. A Machine Learning-Based Gradient Boosting Regression Approach for Wind Power Production Forecasting: A Step towards Smart Grid Environments. (2023). LAPSE:2023.34127
Author Affiliations
Singh U: Department of Electrical Engineering, Delhi Technological University, Delhi 110042, India; Maharaja Surajmal Institute of Technology, Delhi 110058, India
Rizwan M: Department of Electrical Engineering, Delhi Technological University, Delhi 110042, India [ORCID]
Alaraj M: Department of Electrical Engineering, College of Engineering, Qassim University, Buraydah 52571, Qassim, Saudi Arabia [ORCID]
Alsaidan I: Department of Electrical Engineering, College of Engineering, Qassim University, Buraydah 52571, Qassim, Saudi Arabia [ORCID]
Rizwan M: Department of Electrical Engineering, Delhi Technological University, Delhi 110042, India [ORCID]
Alaraj M: Department of Electrical Engineering, College of Engineering, Qassim University, Buraydah 52571, Qassim, Saudi Arabia [ORCID]
Alsaidan I: Department of Electrical Engineering, College of Engineering, Qassim University, Buraydah 52571, Qassim, Saudi Arabia [ORCID]
Journal Name
Energies
Volume
14
Issue
16
First Page
5196
Year
2021
Publication Date
2021-08-23
Published Version
ISSN
1996-1073
Version Comments
Original Submission
Other Meta
PII: en14165196, Publication Type: Journal Article
Record Map
Published Article
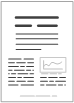
LAPSE:2023.34127
This Record
External Link
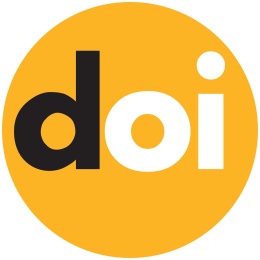
doi:10.3390/en14165196
Publisher Version
Download
Meta
Record Statistics
Record Views
88
Version History
[v1] (Original Submission)
Apr 25, 2023
Verified by curator on
Apr 25, 2023
This Version Number
v1
Citations
Most Recent
This Version
URL Here
https://psecommunity.org/LAPSE:2023.34127
Original Submitter
Auto Uploader for LAPSE
Links to Related Works