LAPSE:2023.3304
Published Article
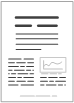
LAPSE:2023.3304
Grid Distribution Fault Occurrence and Remedial Measures Prediction/Forecasting through Different Deep Learning Neural Networks by Using Real Time Data from Tabuk City Power Grid
February 22, 2023
Modern societies need a constant and stable electrical supply. After relying primarily on formal mathematical modeling from operations research, control theory, and numerical analysis, power systems analysis has changed its attention toward AI prediction/forecasting tools. AI techniques have helped fix power system issues in generation, transmission, distribution, scheduling and forecasting, etc. These strategies may assist today’s large power systems which have added more interconnections to meet growing load demands. They make it simple for them to do difficult duties. Identification of problems and problem management have always necessitated the use of labor. These operations are made more sophisticated and data-intensive due to the variety and growth of the networks involved. In light of all of this, the automation of network administration is absolutely necessary. AI has the potential to improve the problem-solving and deductive reasoning approaches used in fault management. This study implements a variety of artificial intelligence and deep learning approaches in order to foresee and predict the corrective measures that will be conducted in response to faults that occur inside the power distribution network of the Grid station in Tabuk city with regard to users. The Tabuk grid station is the source of the data that was gathered for this purpose; it includes a list of defects categorization, actions and remedies that were implemented to overcome these faults, as well as the number of regular and VIP users from 2017 to 2022. Deep learning, the most advanced method of learning used by artificial intelligence, is continuing to make significant strides in a variety of domain areas, including prediction. This study found that the main predictors of remedial measures against the fault occurring in the power systems are the number of customers affected and the actual cause of the fault. Consequently, the deep learning regression model, i.e., Gated Recurrent Unit (GRU), achieved the best performance among the three, which yielded an accuracy of 92.13%, mean absolute error (MAE) loss of 0.37%, and root mean square error (RMSE) loss of 0.39% while the simple RNN model’s performance is not up to the mark with an accuracy of 89.21%, mean absolute error (MAE) loss of 0.45% and root mean square error (RMSE) loss of 0.34%. Significance of the research is to provide the maximum benefit to the customers and the company by using different AI techniques.
Record ID
Keywords
deep learning, fault classification, neural networks, power systems
Subject
Suggested Citation
Almasoudi FM. Grid Distribution Fault Occurrence and Remedial Measures Prediction/Forecasting through Different Deep Learning Neural Networks by Using Real Time Data from Tabuk City Power Grid. (2023). LAPSE:2023.3304
Author Affiliations
Almasoudi FM: Department of Electrical Engineering, Faculty of Engineering, University of Tabuk, Tabuk 47913, Saudi Arabia [ORCID]
Journal Name
Energies
Volume
16
Issue
3
First Page
1026
Year
2023
Publication Date
2023-01-17
Published Version
ISSN
1996-1073
Version Comments
Original Submission
Other Meta
PII: en16031026, Publication Type: Journal Article
Record Map
Published Article
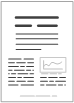
LAPSE:2023.3304
This Record
External Link
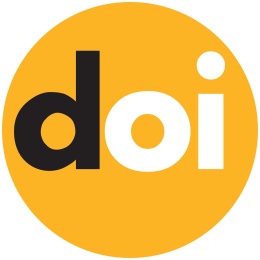
doi:10.3390/en16031026
Publisher Version
Download
Meta
Record Statistics
Record Views
91
Version History
[v1] (Original Submission)
Feb 22, 2023
Verified by curator on
Feb 22, 2023
This Version Number
v1
Citations
Most Recent
This Version
URL Here
https://psecommunity.org/LAPSE:2023.3304
Original Submitter
Auto Uploader for LAPSE
Links to Related Works