LAPSE:2023.32574
Published Article
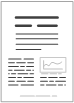
LAPSE:2023.32574
Machine Learning Techniques for Energy Efficiency and Anomaly Detection in Hybrid Wireless Sensor Networks
April 20, 2023
Wireless sensor networks (WSNs) are among the most popular wireless technologies for sensor communication purposes nowadays. Usually, WSNs are developed for specific applications, either monitoring purposes or tracking purposes, for indoor or outdoor environments, where limited battery power is a main challenge. To overcome this problem, many routing protocols have been proposed through the last few years. Nevertheless, the extension of the network lifetime in consideration of the sensors capacities remains an open issue. In this paper, to achieve more efficient and reliable protocols according to current application scenarios, two well-known energy efficient protocols, i.e., Low-Energy Adaptive Clustering hierarchy (LEACH) and Energy−Efficient Sensor Routing (EESR), are redesigned considering neural networks. Specifically, to improve results in terms of energy efficiency, a Levenberg−Marquardt neural network (LMNN) is integrated. Furthermore, in order to improve the performance, a sub-cluster LEACH-derived protocol is also proposed. Simulation results show that the Sub-LEACH with LMNN outperformed its competitors in energy efficiency. In addition, the end-to-end delay was evaluated, and Sub-LEACH protocol proved to be the best among existing strategies. Moreover, an intrusion detection system (IDS) has been proposed for anomaly detection based on the support vector machine (SVM) approach for optimal feature selection. Results showed a 96.15% accuracy—again outperforming existing IDS models. Therefore, satisfactory results in terms of energy efficiency, end-to-end delay and anomaly detection analysis were attained.
Record ID
Keywords
EESR protocol, end-to-end delay, Energy Efficiency, intrusion detection system, LEACH protocol, neural networks, support vector machine
Suggested Citation
Mittal M, de Prado RP, Kawai Y, Nakajima S, Muñoz-Expósito JE. Machine Learning Techniques for Energy Efficiency and Anomaly Detection in Hybrid Wireless Sensor Networks. (2023). LAPSE:2023.32574
Author Affiliations
Mittal M: Centre de Recherche en Informatique, Signal et Automatique de Lille, INRIA, 59655 Villeneuve-d’Ascq, France [ORCID]
de Prado RP: Telecommunication Engineering Department, University of Jaén, 23071 Jaén, Spain [ORCID]
Kawai Y: Department of Information Science and Engineering, Kyoto Sangyo University, Kamingamo, Kita-ku, Kyoto 603-8555, Japan; Cybermedia Center (CMC), Osaka University, Osaka 565-0871, Japan
Nakajima S: Department of Information Science and Engineering, Kyoto Sangyo University, Kamingamo, Kita-ku, Kyoto 603-8555, Japan
Muñoz-Expósito JE: Telecommunication Engineering Department, University of Jaén, 23071 Jaén, Spain [ORCID]
de Prado RP: Telecommunication Engineering Department, University of Jaén, 23071 Jaén, Spain [ORCID]
Kawai Y: Department of Information Science and Engineering, Kyoto Sangyo University, Kamingamo, Kita-ku, Kyoto 603-8555, Japan; Cybermedia Center (CMC), Osaka University, Osaka 565-0871, Japan
Nakajima S: Department of Information Science and Engineering, Kyoto Sangyo University, Kamingamo, Kita-ku, Kyoto 603-8555, Japan
Muñoz-Expósito JE: Telecommunication Engineering Department, University of Jaén, 23071 Jaén, Spain [ORCID]
Journal Name
Energies
Volume
14
Issue
11
First Page
3125
Year
2021
Publication Date
2021-05-27
Published Version
ISSN
1996-1073
Version Comments
Original Submission
Other Meta
PII: en14113125, Publication Type: Journal Article
Record Map
Published Article
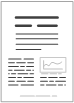
LAPSE:2023.32574
This Record
External Link
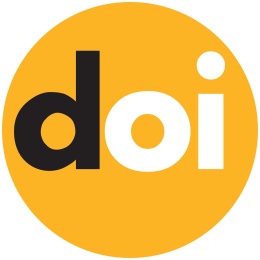
doi:10.3390/en14113125
Publisher Version
Download
Meta
Record Statistics
Record Views
78
Version History
[v1] (Original Submission)
Apr 20, 2023
Verified by curator on
Apr 20, 2023
This Version Number
v1
Citations
Most Recent
This Version
URL Here
https://psecommunity.org/LAPSE:2023.32574
Original Submitter
Auto Uploader for LAPSE
Links to Related Works