LAPSE:2023.30917
Published Article
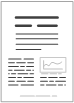
LAPSE:2023.30917
Renewable Scenario Generation Based on the Hybrid Genetic Algorithm with Variable Chromosome Length
April 17, 2023
Determining the operation scenarios of renewable energies is important for power system dispatching. This paper proposes a renewable scenario generation method based on the hybrid genetic algorithm with variable chromosome length (HGAVCL). The discrete wavelet transform (DWT) is used to divide the original data into linear and fluctuant parts according to the length of time scales. The HGAVCL is designed to optimally divide the linear part into different time sections. Additionally, each time section is described by the autoregressive integrated moving average (ARIMA) model. With the consideration of temporal correlation, the Copula joint probability density function is established to model the fluctuant part. Based on the attained ARIMA model and joint probability density function, a number of data are generated by the Monte Carlo method, and the time autocorrelation, average offset rate, and climbing similarity indexes are established to assess the data quality of generated scenarios. A case study is conducted to verify the effectiveness of the proposed approach. The calculated time autocorrelation, average offset rate, and climbing similarity are 0.0515, 0.0396, and 0.9035, respectively, which shows the superior performance of the proposed approach.
Record ID
Keywords
ARIMA model, copula function, Genetic Algorithm, Renewable and Sustainable Energy, scenario generation
Subject
Suggested Citation
Liu X, Wang L, Cao Y, Ma R, Wang Y, Li C, Liu R, Zou S. Renewable Scenario Generation Based on the Hybrid Genetic Algorithm with Variable Chromosome Length. (2023). LAPSE:2023.30917
Author Affiliations
Liu X: Economic and Technological Research Institute of State Grid Shandong Electric Power Company, Jinan 250061, China
Wang L: State Grid Shandong Electric Power Company, Jinan 250001, China
Cao Y: Academy of Intelligent Innovation, Shandong University, Jinan 250101, China; Key Laboratory of Power System Intelligent Dispatch and Control of the Ministry of Education, Shandong University, Jinan 250061, China [ORCID]
Ma R: Key Laboratory of Power System Intelligent Dispatch and Control of the Ministry of Education, Shandong University, Jinan 250061, China
Wang Y: Economic and Technological Research Institute of State Grid Shandong Electric Power Company, Jinan 250061, China
Li C: Key Laboratory of Power System Intelligent Dispatch and Control of the Ministry of Education, Shandong University, Jinan 250061, China
Liu R: Economic and Technological Research Institute of State Grid Shandong Electric Power Company, Jinan 250061, China
Zou S: Key Laboratory of Power System Intelligent Dispatch and Control of the Ministry of Education, Shandong University, Jinan 250061, China
Wang L: State Grid Shandong Electric Power Company, Jinan 250001, China
Cao Y: Academy of Intelligent Innovation, Shandong University, Jinan 250101, China; Key Laboratory of Power System Intelligent Dispatch and Control of the Ministry of Education, Shandong University, Jinan 250061, China [ORCID]
Ma R: Key Laboratory of Power System Intelligent Dispatch and Control of the Ministry of Education, Shandong University, Jinan 250061, China
Wang Y: Economic and Technological Research Institute of State Grid Shandong Electric Power Company, Jinan 250061, China
Li C: Key Laboratory of Power System Intelligent Dispatch and Control of the Ministry of Education, Shandong University, Jinan 250061, China
Liu R: Economic and Technological Research Institute of State Grid Shandong Electric Power Company, Jinan 250061, China
Zou S: Key Laboratory of Power System Intelligent Dispatch and Control of the Ministry of Education, Shandong University, Jinan 250061, China
Journal Name
Energies
Volume
16
Issue
7
First Page
3180
Year
2023
Publication Date
2023-03-31
Published Version
ISSN
1996-1073
Version Comments
Original Submission
Other Meta
PII: en16073180, Publication Type: Journal Article
Record Map
Published Article
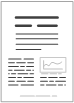
LAPSE:2023.30917
This Record
External Link
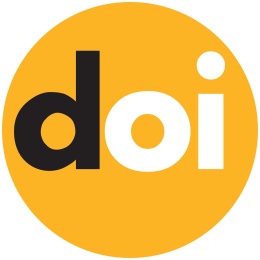
doi:10.3390/en16073180
Publisher Version
Download
Meta
Record Statistics
Record Views
94
Version History
[v1] (Original Submission)
Apr 17, 2023
Verified by curator on
Apr 17, 2023
This Version Number
v1
Citations
Most Recent
This Version
URL Here
https://psecommunity.org/LAPSE:2023.30917
Original Submitter
Auto Uploader for LAPSE
Links to Related Works