LAPSE:2023.30056
Published Article
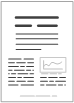
LAPSE:2023.30056
A Long Short-Term Memory Neural Network for the Low-Cost Prediction of Soot Concentration in a Time-Dependent Flame
April 14, 2023
Particulate matter (soot) emissions from combustion processes have damaging health and environmental effects. Numerical techniques with varying levels of accuracy and computational time have been developed to model soot formation in flames. High-fidelity soot models come with a significant computational cost and as a result, accurate soot modelling becomes numerically prohibitive for simulations of industrial combustion devices. In the present study, an accurate and computationally inexpensive soot-estimating tool has been developed using a long short-term memory (LSTM) neural network. The LSTM network is used to estimate the soot volume fraction (fv) in a time-varying, laminar, ethylene/air coflow diffusion flame with 20 Hz periodic fluctuation on the fuel velocity and a 50% amplitude of modulation. The LSTM neural network is trained using data from CFD, where the network inputs are gas properties that are known to impact soot formation (such as temperature) and the network output is fv. The LSTM is shown to give accurate estimations of fv, achieving an average error (relative to CFD) in the peak fv of approximately 30% for the training data and 22% for the test data, all in a computational time that is orders-of-magnitude less than that of high-fidelity CFD modelling. The neural network approach shows great potential to be applied in industrial applications because it can accurately estimate the soot characteristics without the need to solve the soot-related terms and equations.
Record ID
Keywords
Computational Fluid Dynamics, estimator, LSTM, neural network, soot concentration, transient diffusion flame
Subject
Suggested Citation
Jadidi M, Di Liddo L, Dworkin SB. A Long Short-Term Memory Neural Network for the Low-Cost Prediction of Soot Concentration in a Time-Dependent Flame. (2023). LAPSE:2023.30056
Author Affiliations
Jadidi M: Department of Mechanical and Industrial Engineering, Ryerson University, Toronto, ON M5B 2K3, Canada
Di Liddo L: Department of Mechanical and Industrial Engineering, Ryerson University, Toronto, ON M5B 2K3, Canada
Dworkin SB: Department of Mechanical and Industrial Engineering, Ryerson University, Toronto, ON M5B 2K3, Canada
Di Liddo L: Department of Mechanical and Industrial Engineering, Ryerson University, Toronto, ON M5B 2K3, Canada
Dworkin SB: Department of Mechanical and Industrial Engineering, Ryerson University, Toronto, ON M5B 2K3, Canada
Journal Name
Energies
Volume
14
Issue
5
First Page
1394
Year
2021
Publication Date
2021-03-03
Published Version
ISSN
1996-1073
Version Comments
Original Submission
Other Meta
PII: en14051394, Publication Type: Journal Article
Record Map
Published Article
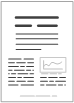
LAPSE:2023.30056
This Record
External Link
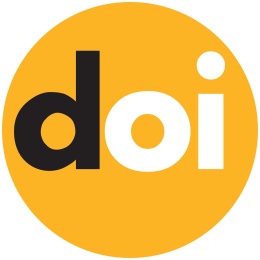
doi:10.3390/en14051394
Publisher Version
Download
Meta
Record Statistics
Record Views
93
Version History
[v1] (Original Submission)
Apr 14, 2023
Verified by curator on
Apr 14, 2023
This Version Number
v1
Citations
Most Recent
This Version
URL Here
https://psecommunity.org/LAPSE:2023.30056
Original Submitter
Auto Uploader for LAPSE
Links to Related Works