LAPSE:2023.29613
Published Article
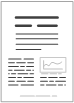
LAPSE:2023.29613
Prediction of Dead Oil Viscosity: Machine Learning vs. Classical Correlations
April 13, 2023
Dead oil viscosity is a critical parameter to solve numerous reservoir engineering problems and one of the most unreliable properties to predict with classical black oil correlations. Determination of dead oil viscosity by experiments is expensive and time-consuming, which means developing an accurate and quick prediction model is required. This paper implements six machine learning models: random forest (RF), lightgbm, XGBoost, multilayer perceptron (MLP) neural network, stochastic real-valued (SRV) and SuperLearner to predict dead oil viscosity. More than 2000 pressure−volume−temperature (PVT) data were used for developing and testing these models. A huge range of viscosity data were used, from light intermediate to heavy oil. In this study, we give insight into the performance of different functional forms that have been used in the literature to formulate dead oil viscosity. The results show that the functional form f(γAPI,T), has the best performance, and additional correlating parameters might be unnecessary. Furthermore, SuperLearner outperformed other machine learning (ML) algorithms as well as common correlations that are based on the metric analysis. The SuperLearner model can potentially replace the empirical models for viscosity predictions on a wide range of viscosities (any oil type). Ultimately, the proposed model is capable of simulating the true physical trend of the dead oil viscosity with variations of oil API gravity, temperature and shear rate.
Record ID
Keywords
dead oil viscosity, Machine Learning, PVT properties, SuperLearner, viscosity
Subject
Suggested Citation
Hadavimoghaddam F, Ostadhassan M, Heidaryan E, Sadri MA, Chapanova I, Popov E, Cheremisin A, Rafieepour S. Prediction of Dead Oil Viscosity: Machine Learning vs. Classical Correlations. (2023). LAPSE:2023.29613
Author Affiliations
Hadavimoghaddam F: Department of Oil Field Development and Operation, Faculty of Oil and Gas Field Development, 119991 Moscow, Russia
Ostadhassan M: Key Laboratory of Continental Shale Hydrocarbon Accumulation and Efficient Development, Ministry of Education, Northeast Petroleum University, Daqing 163318, China [ORCID]
Heidaryan E: Department of Chemical Engineering, Engineering School, University of São Paulo (USP), Caixa Postal 61548, São Paulo 05424-970, Brazil
Sadri MA: Skolkovo Institute of Science and Technology (Skoltech), 143026 Moscow, Russia [ORCID]
Chapanova I: Skolkovo Institute of Science and Technology (Skoltech), 143026 Moscow, Russia
Popov E: Skolkovo Institute of Science and Technology (Skoltech), 143026 Moscow, Russia
Cheremisin A: Skolkovo Institute of Science and Technology (Skoltech), 143026 Moscow, Russia [ORCID]
Rafieepour S: McDougall School of Petroleum Engineering, University of Tulsa, Tulsa, OK 74110, USA
Ostadhassan M: Key Laboratory of Continental Shale Hydrocarbon Accumulation and Efficient Development, Ministry of Education, Northeast Petroleum University, Daqing 163318, China [ORCID]
Heidaryan E: Department of Chemical Engineering, Engineering School, University of São Paulo (USP), Caixa Postal 61548, São Paulo 05424-970, Brazil
Sadri MA: Skolkovo Institute of Science and Technology (Skoltech), 143026 Moscow, Russia [ORCID]
Chapanova I: Skolkovo Institute of Science and Technology (Skoltech), 143026 Moscow, Russia
Popov E: Skolkovo Institute of Science and Technology (Skoltech), 143026 Moscow, Russia
Cheremisin A: Skolkovo Institute of Science and Technology (Skoltech), 143026 Moscow, Russia [ORCID]
Rafieepour S: McDougall School of Petroleum Engineering, University of Tulsa, Tulsa, OK 74110, USA
Journal Name
Energies
Volume
14
Issue
4
First Page
930
Year
2021
Publication Date
2021-02-10
Published Version
ISSN
1996-1073
Version Comments
Original Submission
Other Meta
PII: en14040930, Publication Type: Journal Article
Record Map
Published Article
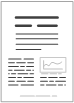
LAPSE:2023.29613
This Record
External Link
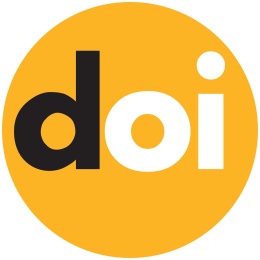
doi:10.3390/en14040930
Publisher Version
Download
Meta
Record Statistics
Record Views
72
Version History
[v1] (Original Submission)
Apr 13, 2023
Verified by curator on
Apr 13, 2023
This Version Number
v1
Citations
Most Recent
This Version
URL Here
https://psecommunity.org/LAPSE:2023.29613
Original Submitter
Auto Uploader for LAPSE
Links to Related Works