LAPSE:2023.28993
Published Article
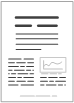
LAPSE:2023.28993
Construction of a Frequency Compliant Unit Commitment Framework Using an Ensemble Learning Technique
April 12, 2023
Frequency control is essential to ensure reliability and quality of power systems. North American Electric Reliability Corporation’s (NERC) Control Performance Standard 1 (CPS1) is widely adopted by many operating authorities to examine the quality of the frequency control. The operating authority would have a strong interest in knowing how the frequency-sensitive features affect the CPS1 score and finding out more effective unit-dispatch schedules for reaching the CPS1 goal. As frequency-sensitive features usually possess multi-variable and high-correlated characteristics, this paper employed an ensemble learning technique (the Gradient Boosting Decision Tree algorithm, GBDT) to construct Frequency Response Model (FRM) of the Taipower system in Taiwan to evaluate by CPS1 score. The proposed CPS1 model was then integrated with Unit Commitment (UC) program to determine the unit-dispatch that achieves the targeted CPS1 score. The feasibility and effectiveness of the proposed CPS1-UC platform were validated and compared with the other benchmark model-based UC methods by two operating cases. The proposed model shows promising results: the system frequency could be maintained well, especially in the periods of the early morning or the high renewable penetration.
Record ID
Keywords
Control Performance Standard 1 (CPS1), frequency control, Machine Learning, unit commitment
Subject
Suggested Citation
Chiu HW, Chang-Chien LR, Wu CC. Construction of a Frequency Compliant Unit Commitment Framework Using an Ensemble Learning Technique. (2023). LAPSE:2023.28993
Author Affiliations
Chiu HW: Department of Electrical Engineering, National Cheng Kung University, East District, Tainan City 701, Taiwan [ORCID]
Chang-Chien LR: Department of Electrical Engineering, National Cheng Kung University, East District, Tainan City 701, Taiwan [ORCID]
Wu CC: Taiwan Power Company, Taipei 10016, Taiwan
Chang-Chien LR: Department of Electrical Engineering, National Cheng Kung University, East District, Tainan City 701, Taiwan [ORCID]
Wu CC: Taiwan Power Company, Taipei 10016, Taiwan
Journal Name
Energies
Volume
14
Issue
2
Article Number
en14020310
Year
2021
Publication Date
2021-01-08
Published Version
ISSN
1996-1073
Version Comments
Original Submission
Other Meta
PII: en14020310, Publication Type: Journal Article
Record Map
Published Article
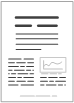
LAPSE:2023.28993
This Record
External Link
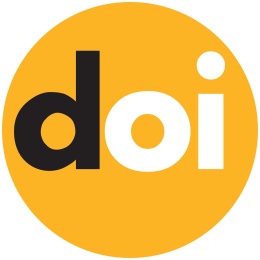
doi:10.3390/en14020310
Publisher Version
Download
Meta
Record Statistics
Record Views
73
Version History
[v1] (Original Submission)
Apr 12, 2023
Verified by curator on
Apr 12, 2023
This Version Number
v1
Citations
Most Recent
This Version
URL Here
https://psecommunity.org/LAPSE:2023.28993
Original Submitter
Auto Uploader for LAPSE
Links to Related Works