LAPSE:2023.27439
Published Article
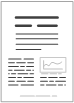
LAPSE:2023.27439
Data Mining and Machine Learning Techniques for Aerodynamic Databases: Introduction, Methodology and Potential Benefits
April 4, 2023
Machine learning and data mining techniques are nowadays being used in many business sectors to exploit the data in order to detect trends, discover certain features and patters, or even predict the future. However, in the field of aerodynamics, the application of these techniques is still in the initial stages. This paper focuses on exploring the benefits that machine learning and data mining techniques can offer to aerodynamicists in order to extract knowledge from the CFD data and to make quick predictions of aerodynamic coefficients. For this purpose, three aerodynamic databases (NACA0012 airfoil, RAE2822 airfoil and 3D DPW wing) have been used and results show that machine-learning and data-mining techniques have a huge potential also in this field.
Record ID
Keywords
aerodynamic analysis, Computational Fluid Dynamics, data mining, linear regression, Machine Learning, support vector regression, surrogate modeling
Subject
Suggested Citation
Andrés-Pérez E. Data Mining and Machine Learning Techniques for Aerodynamic Databases: Introduction, Methodology and Potential Benefits. (2023). LAPSE:2023.27439
Author Affiliations
Andrés-Pérez E: Theoretical and Computational Aerodynamics Branch, Flight Physics Department, Spanish National Institute for Aerospace Technology (INTA), Ctra. Ajalvir, km. 4, 28850 Torrejón de Ardoz, Spain [ORCID]
Journal Name
Energies
Volume
13
Issue
21
Article Number
E5807
Year
2020
Publication Date
2020-11-06
Published Version
ISSN
1996-1073
Version Comments
Original Submission
Other Meta
PII: en13215807, Publication Type: Journal Article
Record Map
Published Article
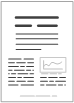
LAPSE:2023.27439
This Record
External Link
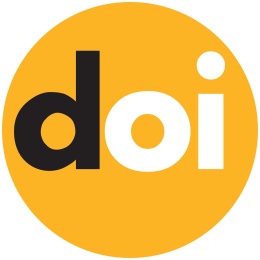
doi:10.3390/en13215807
Publisher Version
Download
Meta
Record Statistics
Record Views
77
Version History
[v1] (Original Submission)
Apr 4, 2023
Verified by curator on
Apr 4, 2023
This Version Number
v1
Citations
Most Recent
This Version
URL Here
https://psecommunity.org/LAPSE:2023.27439
Original Submitter
Auto Uploader for LAPSE
Links to Related Works