LAPSE:2023.26105
Published Article
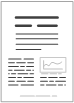
LAPSE:2023.26105
Method for Clustering Daily Load Curve Based on SVD-KICIC
March 31, 2023
Clustering electric load curves is an important part of the load data mining process. In this paper, we propose a clustering algorithm by combining singular value decomposition and KICIC clustering algorithm (SVD-KICIC) for analyzing the characteristics of daily load curves to mitigate some of the traditional clustering algorithm problems, such as only considering intra-class distance and low computational efficiency when dealing with massive load data. Our method identifies effective daily load curve characteristics using the singular value decomposition technique to improve dimensionality reduction, which improves low computational efficiency by reducing the number of dimensions inherent in big data. Additionally, the method performs SVD on the load data to obtain singular values for determination of weight of the KICIC algorithm, which leverages intra-class and inter-class distances of the load data and further improves the computational efficiency of the algorithm. Finally, we perform a series of simulations of actual load curves from a certain city to validate that the algorithm proposed in this paper has a short operation time, high clustering quality, and solid robustness that improves the clustering performance of the load curves.
Record ID
Keywords
classification of load curves, clustering validity, dimensionality reduction, inter-class distance, singular value decomposition, weighted Euclidean distance
Subject
Suggested Citation
Zhang Y, Zhang J, Yao G, Xu X, Wei K. Method for Clustering Daily Load Curve Based on SVD-KICIC. (2023). LAPSE:2023.26105
Author Affiliations
Zhang Y: School of Electrical Engineering, Guizhou University, Guiyang 550025, China
Zhang J: School of Electrical Engineering, Guizhou University, Guiyang 550025, China [ORCID]
Yao G: Guizhou Power Grid Company, Guiyang 550001, China
Xu X: School of Electrical Engineering, Guizhou University, Guiyang 550025, China
Wei K: School of Electrical Engineering, Guizhou University, Guiyang 550025, China
Zhang J: School of Electrical Engineering, Guizhou University, Guiyang 550025, China [ORCID]
Yao G: Guizhou Power Grid Company, Guiyang 550001, China
Xu X: School of Electrical Engineering, Guizhou University, Guiyang 550025, China
Wei K: School of Electrical Engineering, Guizhou University, Guiyang 550025, China
Journal Name
Energies
Volume
13
Issue
17
Article Number
E4476
Year
2020
Publication Date
2020-08-31
Published Version
ISSN
1996-1073
Version Comments
Original Submission
Other Meta
PII: en13174476, Publication Type: Journal Article
Record Map
Published Article
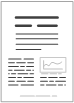
LAPSE:2023.26105
This Record
External Link
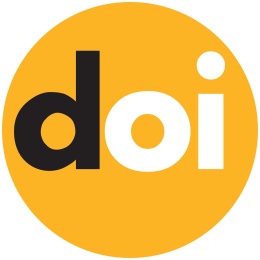
doi:10.3390/en13174476
Publisher Version
Download
Meta
Record Statistics
Record Views
91
Version History
[v1] (Original Submission)
Mar 31, 2023
Verified by curator on
Mar 31, 2023
This Version Number
v1
Citations
Most Recent
This Version
URL Here
https://psecommunity.org/LAPSE:2023.26105
Original Submitter
Auto Uploader for LAPSE
Links to Related Works