LAPSE:2023.20003
Published Article
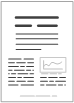
LAPSE:2023.20003
A Simulation Environment for Training a Reinforcement Learning Agent Trading a Battery Storage
March 10, 2023
Battery storages are an essential element of the emerging smart grid. Compared to other distributed intelligent energy resources, batteries have the advantage of being able to rapidly react to events such as renewable generation fluctuations or grid disturbances. There is a lack of research on ways to profitably exploit this ability. Any solution needs to consider rapid electrical phenomena as well as the much slower dynamics of relevant electricity markets. Reinforcement learning is a branch of artificial intelligence that has shown promise in optimizing complex problems involving uncertainty. This article applies reinforcement learning to the problem of trading batteries. The problem involves two timescales, both of which are important for profitability. Firstly, trading the battery capacity must occur on the timescale of the chosen electricity markets. Secondly, the real-time operation of the battery must ensure that no financial penalties are incurred from failing to meet the technical specification. The trading-related decisions must be done under uncertainties, such as unknown future market prices and unpredictable power grid disturbances. In this article, a simulation model of a battery system is proposed as the environment to train a reinforcement learning agent to make such decisions. The system is demonstrated with an application of the battery to Finnish primary frequency reserve markets.
Record ID
Keywords
Artificial Intelligence, battery, electricity market, frequency containment reserve, frequency reserve, real-time, reinforcement learning, Simulation, timescale
Subject
Suggested Citation
Aaltonen H, Sierla S, Subramanya R, Vyatkin V. A Simulation Environment for Training a Reinforcement Learning Agent Trading a Battery Storage. (2023). LAPSE:2023.20003
Author Affiliations
Aaltonen H: Department of Electrical Engineering and Automation, School of Electrical Engineering, Aalto University, FI-00076 Espoo, Finland [ORCID]
Sierla S: Department of Electrical Engineering and Automation, School of Electrical Engineering, Aalto University, FI-00076 Espoo, Finland [ORCID]
Subramanya R: Department of Electrical Engineering and Automation, School of Electrical Engineering, Aalto University, FI-00076 Espoo, Finland [ORCID]
Vyatkin V: Department of Electrical Engineering and Automation, School of Electrical Engineering, Aalto University, FI-00076 Espoo, Finland; Department of Computer Science, Electrical and Space Engineering, LuleƄ University of Technology, 97187 LuleƄ, Sweden; Inte
Sierla S: Department of Electrical Engineering and Automation, School of Electrical Engineering, Aalto University, FI-00076 Espoo, Finland [ORCID]
Subramanya R: Department of Electrical Engineering and Automation, School of Electrical Engineering, Aalto University, FI-00076 Espoo, Finland [ORCID]
Vyatkin V: Department of Electrical Engineering and Automation, School of Electrical Engineering, Aalto University, FI-00076 Espoo, Finland; Department of Computer Science, Electrical and Space Engineering, LuleƄ University of Technology, 97187 LuleƄ, Sweden; Inte
Journal Name
Energies
Volume
14
Issue
17
First Page
5587
Year
2021
Publication Date
2021-09-06
Published Version
ISSN
1996-1073
Version Comments
Original Submission
Other Meta
PII: en14175587, Publication Type: Journal Article
Record Map
Published Article
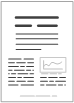
LAPSE:2023.20003
This Record
External Link
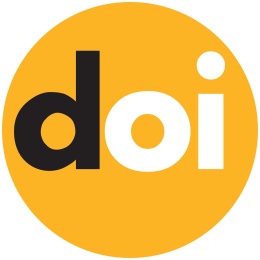
doi:10.3390/en14175587
Publisher Version
Download
Meta
Record Statistics
Record Views
102
Version History
[v1] (Original Submission)
Mar 10, 2023
Verified by curator on
Mar 10, 2023
This Version Number
v1
Citations
Most Recent
This Version
URL Here
https://psecommunity.org/LAPSE:2023.20003
Original Submitter
Auto Uploader for LAPSE
Links to Related Works