LAPSE:2023.19140
Published Article
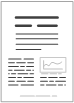
LAPSE:2023.19140
Forecasting the CO2 Emissions at the Global Level: A Multilayer Artificial Neural Network Modelling
March 9, 2023
Better accuracy in short-term forecasting is required for intermediate planning for the national target to reduce CO2 emissions. High stake climate change conventions need accurate predictions of the future emission growth path of the participating countries to make informed decisions. The current study forecasts the CO2 emissions of the 17 key emitting countries. Unlike previous studies where linear statistical modeling is used to forecast the emissions, we develop a multilayer artificial neural network model to forecast the emissions. This model is a dynamic nonlinear model that helps to obtain optimal weights for the predictors with a high level of prediction accuracy. The model uses the gross domestic product (GDP), urban population ratio, and trade openness, as predictors for CO2 emissions. We observe an average of 96% prediction accuracy among the 17 countries which is much higher than the accuracy of the previous models. Using the optimal weights and available input data the forecasting of CO2 emissions is undertaken. The results show that high emitting countries, such as China, India, Iran, Indonesia, and Saudi Arabia are expected to increase their emissions in the near future. Currently, low emitting countries, such as Brazil, South Africa, Turkey, and South Korea will also tread on a high emission growth path. On the other hand, the USA, Japan, UK, France, Italy, Australia, and Canada will continuously reduce their emissions. These findings will help the countries to engage in climate mitigation and adaptation negotiations.
Record ID
Keywords
artificial neural network model, CO2 emission, forecasting, Simulation
Suggested Citation
Jena PR, Managi S, Majhi B. Forecasting the CO2 Emissions at the Global Level: A Multilayer Artificial Neural Network Modelling. (2023). LAPSE:2023.19140
Author Affiliations
Jena PR: School of Management, National Institute of Technology Karnataka, Surathkal, Mangalore 575025, India [ORCID]
Managi S: Urban Institute & Department of Civil Engineering, Kyushu University, Fukuoka 819-0395, Japan
Majhi B: Department of CSIT, Guru Ghasidas Vishwavidyalaya, Central University, Bilaspur 495009, India [ORCID]
Managi S: Urban Institute & Department of Civil Engineering, Kyushu University, Fukuoka 819-0395, Japan
Majhi B: Department of CSIT, Guru Ghasidas Vishwavidyalaya, Central University, Bilaspur 495009, India [ORCID]
Journal Name
Energies
Volume
14
Issue
19
First Page
6336
Year
2021
Publication Date
2021-10-04
Published Version
ISSN
1996-1073
Version Comments
Original Submission
Other Meta
PII: en14196336, Publication Type: Journal Article
Record Map
Published Article
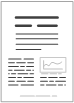
LAPSE:2023.19140
This Record
External Link
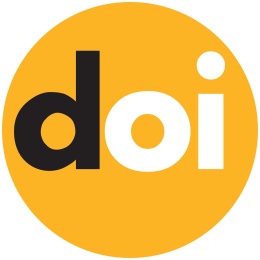
doi:10.3390/en14196336
Publisher Version
Download
Meta
Record Statistics
Record Views
64
Version History
[v1] (Original Submission)
Mar 9, 2023
Verified by curator on
Mar 9, 2023
This Version Number
v1
Citations
Most Recent
This Version
URL Here
https://psecommunity.org/LAPSE:2023.19140
Original Submitter
Auto Uploader for LAPSE
Links to Related Works