LAPSE:2023.15903
Published Article
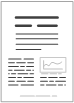
LAPSE:2023.15903
Inter-Hour Forecast of Solar Radiation Based on Long Short-Term Memory with Attention Mechanism and Genetic Algorithm
March 2, 2023
The installed capacity of photovoltaic power generation occupies an increasing proportion in the power system, and its stability is greatly affected by the fluctuation of solar radiation. Accurate prediction of solar radiation is an important prerequisite for ensuring power grid security and electricity market transactions. The current mainstream solar radiation prediction method is the deep learning method, and the structure design and data selection of the deep learning method determine the prediction accuracy and speed of the network. In this paper, we propose a novel long short-term memory (LSTM) model based on the attention mechanism and genetic algorithm (AGA-LSTM). The attention mechanism is used to assign different weights to each feature, so that the model can focus more attention on the key features. Meanwhile, the structure and data selection parameters of the model are optimized through genetic algorithms, and the time series memory and processing capabilities of LSTM are used to predict the global horizontal irradiance and direct normal irradiance after 5, 10, and 15 min. The proposed AGA-LSTM model was trained and tested with two years of data from the public database Solar Radiation Research Laboratory site of the National Renewable Energy Laboratory. The experimental results show that under the three prediction scales, the prediction performance of the AGA-LSTM model is below 20%, which effectively improves the prediction accuracy compared with the continuous model and some public methods.
Record ID
Keywords
attention mechanism, Genetic Algorithm, inter-hour forecast, long short-term memory, solar radiation
Subject
Suggested Citation
Zhu T, Li Y, Li Z, Guo Y, Ni C. Inter-Hour Forecast of Solar Radiation Based on Long Short-Term Memory with Attention Mechanism and Genetic Algorithm. (2023). LAPSE:2023.15903
Author Affiliations
Zhu T: College of Mechanical and Electronic Engineering, Nanjing Forestry University, Nanjing 210037, China; Key Laboratory of Measurement and Control of Complex Systems of Engineering, Southeast University, Ministry of Education, Nanjing 210096, China [ORCID]
Li Y: College of Mechanical and Electronic Engineering, Nanjing Forestry University, Nanjing 210037, China
Li Z: College of Mechanical and Electronic Engineering, Nanjing Forestry University, Nanjing 210037, China
Guo Y: College of Mechanical and Electronic Engineering, Nanjing Forestry University, Nanjing 210037, China
Ni C: College of Mechanical and Electronic Engineering, Nanjing Forestry University, Nanjing 210037, China
Li Y: College of Mechanical and Electronic Engineering, Nanjing Forestry University, Nanjing 210037, China
Li Z: College of Mechanical and Electronic Engineering, Nanjing Forestry University, Nanjing 210037, China
Guo Y: College of Mechanical and Electronic Engineering, Nanjing Forestry University, Nanjing 210037, China
Ni C: College of Mechanical and Electronic Engineering, Nanjing Forestry University, Nanjing 210037, China
Journal Name
Energies
Volume
15
Issue
3
First Page
1062
Year
2022
Publication Date
2022-01-31
Published Version
ISSN
1996-1073
Version Comments
Original Submission
Other Meta
PII: en15031062, Publication Type: Journal Article
Record Map
Published Article
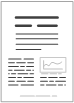
LAPSE:2023.15903
This Record
External Link
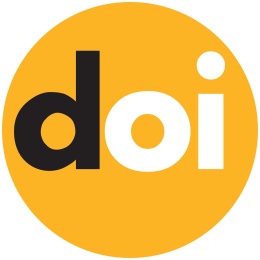
doi:10.3390/en15031062
Publisher Version
Download
Meta
Record Statistics
Record Views
82
Version History
[v1] (Original Submission)
Mar 2, 2023
Verified by curator on
Mar 2, 2023
This Version Number
v1
Citations
Most Recent
This Version
URL Here
https://psecommunity.org/LAPSE:2023.15903
Original Submitter
Auto Uploader for LAPSE
Links to Related Works