LAPSE:2023.14058
Published Article
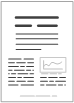
LAPSE:2023.14058
Shapelets to Classify Energy Demand Time Series
March 1, 2023
Data are an important asset that the electric power industry have available today to support management decisions, excel in operational efficiency, and be more competitive. The advent of smart grids has increased power grid sensorization and so, too, the data availability. However, the inability to recognize the value of data beyond the siloed application in which data are collected is seen as a barrier. Power load time series are one of the most important types of data collected by utilities, because of the inherent information in them (e.g., power load time series comprehend human behavior, economic momentum, and other trends). The area of time series analysis in the energy domain is attracting considerable interest because of growing available data as more sensorization is deployed in power grids. This study considers the shapelet technique to create interpretable classifiers for four use cases. The study systematically applied the shapelet technique to data from different hierarchical power levels (national, primary power substations, and secondary power substations). The study has experimentally shown shapelets as a technique that embraces the interpretability and accuracy of the learning models, the ability to extract interpretable patterns and knowledge, and the ability to recognize and monetize the value of the data, important subjects to reinforce the importance of data-driven services within the energy sector.
Record ID
Keywords
electricity demand, Energy, energy digitalization, Machine Learning, shapelets, time series classification
Subject
Suggested Citation
Pinheiro MG, Madeira SC, Francisco AP. Shapelets to Classify Energy Demand Time Series. (2023). LAPSE:2023.14058
Author Affiliations
Pinheiro MG: Instituto Superior Técnico, Universidade de Lisboa, 1049-001 Lisbon, Portugal; EDP, Av. 24 de Julho 12, 1249-300 Lisbon, Portugal [ORCID]
Madeira SC: LASIGE, Faculdade de Ciências, Universidade de Lisboa, 1749-016 Lisbon, Portugal [ORCID]
Francisco AP: Instituto Superior Técnico, Universidade de Lisboa, 1049-001 Lisbon, Portugal; INESC-ID Lisboa, Rua Alves Redol 9, 1000-029 Lisbon, Portugal [ORCID]
Madeira SC: LASIGE, Faculdade de Ciências, Universidade de Lisboa, 1749-016 Lisbon, Portugal [ORCID]
Francisco AP: Instituto Superior Técnico, Universidade de Lisboa, 1049-001 Lisbon, Portugal; INESC-ID Lisboa, Rua Alves Redol 9, 1000-029 Lisbon, Portugal [ORCID]
Journal Name
Energies
Volume
15
Issue
8
First Page
2960
Year
2022
Publication Date
2022-04-18
Published Version
ISSN
1996-1073
Version Comments
Original Submission
Other Meta
PII: en15082960, Publication Type: Journal Article
Record Map
Published Article
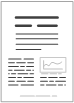
LAPSE:2023.14058
This Record
External Link
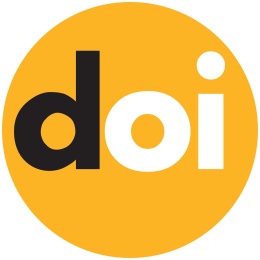
doi:10.3390/en15082960
Publisher Version
Download
Meta
Record Statistics
Record Views
75
Version History
[v1] (Original Submission)
Mar 1, 2023
Verified by curator on
Mar 1, 2023
This Version Number
v1
Citations
Most Recent
This Version
URL Here
https://psecommunity.org/LAPSE:2023.14058
Original Submitter
Auto Uploader for LAPSE
Links to Related Works