LAPSE:2023.11668
Published Article
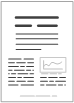
LAPSE:2023.11668
Combining Deep Learning and the Heat Flux Method for In-Situ Thermal-Transmittance Measurement Improvement
February 27, 2023
Transmission losses through the building envelope account for a large proportion of building energy balance. One of the most important parameters for determining transmission losses is thermal transmittance. Although thermal transmittance does not take into account dynamic parameters, it is traditionally the most commonly used estimation of transmission losses due to its simplicity and efficiency. It is challenging to estimate the thermal transmittance of an existing building element because thermal properties are commonly unknown or not all the layers that make up the element can be found due to technical-drawing information loss. In such cases, experimental methods are essential, the most common of which is the heat-flux method (HFM). One of the main drawbacks of the HFM is the long measurement duration. This research presents the application of deep learning on HFM results by applying long-short term memory units on temperature difference and measured heat flux. This deep-learning regression problem predicts heat flux after the applied model is properly trained on temperature-difference input, which is backpropagated by measured heat flux. The paper shows the performance of the developed procedure on real-size walls under the simulated environmental conditions, while the possibility of practical application is shown in pilot in-situ measurements.
Record ID
Keywords
building physics, deep learning, Energy Efficiency, Machine Learning, thermal transmittance
Subject
Suggested Citation
Gumbarević S, Milovanović B, Dalbelo Bašić B, Gaši M. Combining Deep Learning and the Heat Flux Method for In-Situ Thermal-Transmittance Measurement Improvement. (2023). LAPSE:2023.11668
Author Affiliations
Gumbarević S: Ericsson Nikola Tesla, Krapinska 45, 10000 Zagreb, Croatia [ORCID]
Milovanović B: Faculty of Civil Engineering, University of Zagreb, 10000 Zagreb, Croatia [ORCID]
Dalbelo Bašić B: Faculty of Electrical Engineering and Computing, University of Zagreb, 10000 Zagreb, Croatia [ORCID]
Gaši M: Faculty of Civil Engineering, University of Zagreb, 10000 Zagreb, Croatia [ORCID]
Milovanović B: Faculty of Civil Engineering, University of Zagreb, 10000 Zagreb, Croatia [ORCID]
Dalbelo Bašić B: Faculty of Electrical Engineering and Computing, University of Zagreb, 10000 Zagreb, Croatia [ORCID]
Gaši M: Faculty of Civil Engineering, University of Zagreb, 10000 Zagreb, Croatia [ORCID]
Journal Name
Energies
Volume
15
Issue
14
First Page
5029
Year
2022
Publication Date
2022-07-09
Published Version
ISSN
1996-1073
Version Comments
Original Submission
Other Meta
PII: en15145029, Publication Type: Journal Article
Record Map
Published Article
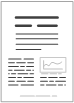
LAPSE:2023.11668
This Record
External Link
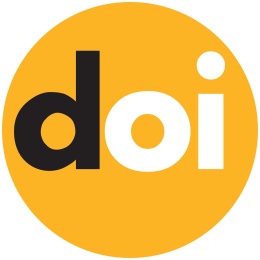
doi:10.3390/en15145029
Publisher Version
Download
Meta
Record Statistics
Record Views
56
Version History
[v1] (Original Submission)
Feb 27, 2023
Verified by curator on
Feb 27, 2023
This Version Number
v1
Citations
Most Recent
This Version
URL Here
https://psecommunity.org/LAPSE:2023.11668
Original Submitter
Auto Uploader for LAPSE
Links to Related Works