LAPSE:2023.11303
Published Article
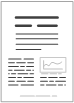
LAPSE:2023.11303
Industrial Application of Data-Driven Process Monitoring with an Automatic Selection Strategy for Modeling Data
February 27, 2023
The increasing scale of industrial processes has significantly motivated the development of data-driven fault detection and diagnosis techniques. The selection of representative fault-free modeling data from operation history is an important prerequisite to establishing a long-term effective process monitoring model. However, industrial data are characterized by a high dimension and multimode, and are also contaminated with both outliers and frequent random disturbances, making automatic modeling data selection a great challenge in industrial applications. In this work, an information entropy-based automatic selection strategy for modeling data is proposed, based on which a general real-time process monitoring framework is developed for a large-scale industrial methanol to olefin unit with multiple operating conditions. Modeling data representing normal operating conditions are automatically selected with only a few manually defined normal samples. A long-term effective process monitoring model is then established based on a multi-layer autoencoder, through which unexpected disturbances in real-time operation can be detected early and the root cause can be preliminarily diagnosed by contribution plots. The adjustment of operating conditions has also been considered through a model update strategy. Details of the proposed data selection strategy and modeling process have been provided to facilitate the industrial application of process monitoring systems by other researchers or companies.
Record ID
Keywords
autoencoder, fault detection and diagnosis, industrial process safety, information entropy, real-time industrial application of process monitoring method
Subject
Suggested Citation
Sun W, Zhou Z, Ma F, Wang J, Ji C. Industrial Application of Data-Driven Process Monitoring with an Automatic Selection Strategy for Modeling Data. (2023). LAPSE:2023.11303
Author Affiliations
Sun W: College of Chemical Engineering, Beijing University of Chemical Technology, North Third Ring Road No.15, Beijing 100029, China [ORCID]
Zhou Z: College of Chemical Engineering, Beijing University of Chemical Technology, North Third Ring Road No.15, Beijing 100029, China
Ma F: College of Chemical Engineering, Beijing University of Chemical Technology, North Third Ring Road No.15, Beijing 100029, China; Center of Process Monitoring and Data Analysis, Wuxi Research Institute of Applied Technologies, Tsinghua University, Wuxi 2140 [ORCID]
Wang J: College of Chemical Engineering, Beijing University of Chemical Technology, North Third Ring Road No.15, Beijing 100029, China [ORCID]
Ji C: College of Chemical Engineering, Beijing University of Chemical Technology, North Third Ring Road No.15, Beijing 100029, China [ORCID]
Zhou Z: College of Chemical Engineering, Beijing University of Chemical Technology, North Third Ring Road No.15, Beijing 100029, China
Ma F: College of Chemical Engineering, Beijing University of Chemical Technology, North Third Ring Road No.15, Beijing 100029, China; Center of Process Monitoring and Data Analysis, Wuxi Research Institute of Applied Technologies, Tsinghua University, Wuxi 2140 [ORCID]
Wang J: College of Chemical Engineering, Beijing University of Chemical Technology, North Third Ring Road No.15, Beijing 100029, China [ORCID]
Ji C: College of Chemical Engineering, Beijing University of Chemical Technology, North Third Ring Road No.15, Beijing 100029, China [ORCID]
Journal Name
Processes
Volume
11
Issue
2
First Page
402
Year
2023
Publication Date
2023-01-28
Published Version
ISSN
2227-9717
Version Comments
Original Submission
Other Meta
PII: pr11020402, Publication Type: Journal Article
Record Map
Published Article
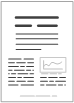
LAPSE:2023.11303
This Record
External Link
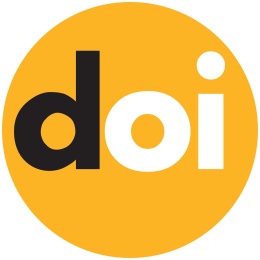
doi:10.3390/pr11020402
Publisher Version
Download
Meta
Record Statistics
Record Views
89
Version History
[v1] (Original Submission)
Feb 27, 2023
Verified by curator on
Feb 27, 2023
This Version Number
v1
Citations
Most Recent
This Version
URL Here
https://psecommunity.org/LAPSE:2023.11303
Original Submitter
Auto Uploader for LAPSE
Links to Related Works