LAPSE:2023.11285
Published Article
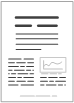
LAPSE:2023.11285
A Novel Fault Detection Method Based on One-Dimension Convolutional Adversarial Autoencoder (1DAAE)
February 27, 2023
Fault detection is an important and demanding problem in industry. Recently, many researchers have addressed the use of deep learning architectures for fault detection applications such as an autoencoder. Traditional methods based on an autoencoder usually complete fault detection by comparing reconstruction errors, and ignore a lot of useful information about the distribution of latent variables. To deal with this problem, this paper proposes a novel unsupervised fault detection method named one-dimension convolutional adversarial autoencoder (1DAAE), which introduces two new ideas: one-dimension convolution layers for the encoder to obtain better features and the adversarial thought to impose the latent variable z to cluster into a prior distribution. The proposed method not only has powerful feature representation ability than the traditional autoencoder, but has also enhanced the discrimination ability by imposing a prior distribution of the latent variables to cluster. Then, two anomaly scores for 1DAAE were proposed to detect fault samples, one based on reconstruction errors, and the other based on latent variable distribution. Finally, it was shown by the experiments that the proposed method outperformed the autoencoder-based, adversarial autoencoder-based, one-dimension convolutional autoencoder-based and generative adversarial network-based algorithms on the Tennessee Eastman process. Through the experiments, we found that the both one-dimension convolution layers and the latent vector distribution are helpful for fault detection.
Record ID
Keywords
autoencoder, convolutional layer, Fault Detection, Tennessee Eastman process, unsupervised learning
Subject
Suggested Citation
Wang J, Li Y, Han Z. A Novel Fault Detection Method Based on One-Dimension Convolutional Adversarial Autoencoder (1DAAE). (2023). LAPSE:2023.11285
Author Affiliations
Journal Name
Processes
Volume
11
Issue
2
First Page
384
Year
2023
Publication Date
2023-01-27
Published Version
ISSN
2227-9717
Version Comments
Original Submission
Other Meta
PII: pr11020384, Publication Type: Journal Article
Record Map
Published Article
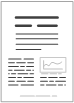
LAPSE:2023.11285
This Record
External Link
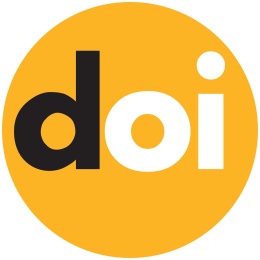
doi:10.3390/pr11020384
Publisher Version
Download
Meta
Record Statistics
Record Views
76
Version History
[v1] (Original Submission)
Feb 27, 2023
Verified by curator on
Feb 27, 2023
This Version Number
v1
Citations
Most Recent
This Version
URL Here
https://psecommunity.org/LAPSE:2023.11285
Original Submitter
Auto Uploader for LAPSE
Links to Related Works