LAPSE:2023.11221
Published Article
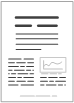
LAPSE:2023.11221
A Three-Step Framework for Multimodal Industrial Process Monitoring Based on DLAN, TSQTA, and FSBN
February 27, 2023
The process monitoring method for industrial production can technically achieve early warning of abnormal situations and help operators make timely and reliable response decisions. Because practical industrial processes have multimodal operating conditions, the data distributions of process variables are different. The different data distributions may cause the fault detection model to be invalid. In addition, the fault diagnosis model cannot find the correct root cause variable of system failure by only identifying abnormal variables. There are correlations between the trend states of the process variables. If we do not consider these correlations, this may result in an incorrect fault root cause. Therefore, multimodal industrial process monitoring is a tough issue. In this paper, we propose a three-step framework for multimodal industrial process monitoring. The framework aims for multimodal industrial processes to detect the faulty status timely and then find the correct root variable that causes the failure. We present deep local adaptive network (DLAN), two-stage qualitative trend analysis (TSQTA), and five-state Bayesian network (FSBN) to implement fault detection, identification, and diagnosis step by step. This framework can detect the system failure timely, identify abnormal variables, and find the root cause variable and the fault propagation path. The case studies on the Tennessee Eastman simulation and a practical chlorobenzene production process are provided to verify the effectiveness of the proposed framework in multimodal industrial process monitoring.
Record ID
Keywords
Bayesian network, deep neural network, industrial safety, multimodality, process monitoring
Subject
Suggested Citation
Wu H, Fu W, Ren X, Wang H, Wang E. A Three-Step Framework for Multimodal Industrial Process Monitoring Based on DLAN, TSQTA, and FSBN. (2023). LAPSE:2023.11221
Author Affiliations
Wu H: China Huaneng Clean Energy Research Institute, Beijing 102209, China [ORCID]
Fu W: Clean Energy Branch of Huaneng (Zhejiang) Energy Development Co., Ltd., Hangzhou 310011, China
Ren X: China Huaneng Clean Energy Research Institute, Beijing 102209, China
Wang H: China Huaneng Clean Energy Research Institute, Beijing 102209, China
Wang E: China Huaneng Clean Energy Research Institute, Beijing 102209, China
Fu W: Clean Energy Branch of Huaneng (Zhejiang) Energy Development Co., Ltd., Hangzhou 310011, China
Ren X: China Huaneng Clean Energy Research Institute, Beijing 102209, China
Wang H: China Huaneng Clean Energy Research Institute, Beijing 102209, China
Wang E: China Huaneng Clean Energy Research Institute, Beijing 102209, China
Journal Name
Processes
Volume
11
Issue
2
First Page
318
Year
2023
Publication Date
2023-01-18
Published Version
ISSN
2227-9717
Version Comments
Original Submission
Other Meta
PII: pr11020318, Publication Type: Journal Article
Record Map
Published Article
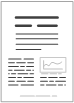
LAPSE:2023.11221
This Record
External Link
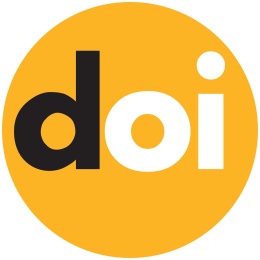
doi:10.3390/pr11020318
Publisher Version
Download
Meta
Record Statistics
Record Views
94
Version History
[v1] (Original Submission)
Feb 27, 2023
Verified by curator on
Feb 27, 2023
This Version Number
v1
Citations
Most Recent
This Version
URL Here
https://psecommunity.org/LAPSE:2023.11221
Original Submitter
Auto Uploader for LAPSE
Links to Related Works