LAPSE:2023.10451
Published Article
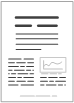
LAPSE:2023.10451
Vibration Fault Detection in Wind Turbines Based on Normal Behaviour Models without Feature Engineering
February 27, 2023
Most wind turbines are remotely monitored 24/7 to allow for early detection of operation problems and developing damage. We present a new fault detection approach for vibration-monitored drivetrains that does not require any feature engineering. Our method relies on a simple model architecture to enable a straightforward implementation in practice. We propose to apply convolutional autoencoders for identifying and extracting the most relevant features from a broad continuous range of the spectrum in an automated manner, saving time and effort. We focus on the range of [0, 1000] Hz for demonstration purposes. A spectral model of the normal vibration response is learnt for the monitored component from past measurements. We demonstrate that the trained model can successfully distinguish damaged from healthy components and detect a damaged generator bearing and damaged gearbox parts from their vibration responses. Using measurements from commercial wind turbines and a test rig, we show that vibration-based fault detection in wind turbine drivetrains can be performed without the usual upfront definition of spectral features. Another advantage of the presented method is that a broad continuous range of the spectrum can be monitored instead of the usual focus on monitoring individual frequencies and harmonics. Future research should investigate the proposed method on more comprehensive datasets and fault types.
Record ID
Keywords
autoencoders, condition monitoring, convolutional autoencoders, Fault Detection, neural networks, Renewable and Sustainable Energy, vibrations, wind turbines
Suggested Citation
Jonas S, Anagnostos D, Brodbeck B, Meyer A. Vibration Fault Detection in Wind Turbines Based on Normal Behaviour Models without Feature Engineering. (2023). LAPSE:2023.10451
Author Affiliations
Jonas S: School of Engineering and Computer Science, Bern University of Applied Sciences, Quellgasse 21, 2501 Biel, Switzerland
Anagnostos D: WinJi AG, Badenerstrasse 808, 8048 Zurich, Switzerland
Brodbeck B: WinJi AG, Badenerstrasse 808, 8048 Zurich, Switzerland
Meyer A: School of Engineering and Computer Science, Bern University of Applied Sciences, Quellgasse 21, 2501 Biel, Switzerland [ORCID]
Anagnostos D: WinJi AG, Badenerstrasse 808, 8048 Zurich, Switzerland
Brodbeck B: WinJi AG, Badenerstrasse 808, 8048 Zurich, Switzerland
Meyer A: School of Engineering and Computer Science, Bern University of Applied Sciences, Quellgasse 21, 2501 Biel, Switzerland [ORCID]
Journal Name
Energies
Volume
16
Issue
4
First Page
1760
Year
2023
Publication Date
2023-02-10
Published Version
ISSN
1996-1073
Version Comments
Original Submission
Other Meta
PII: en16041760, Publication Type: Journal Article
Record Map
Published Article
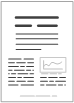
LAPSE:2023.10451
This Record
External Link
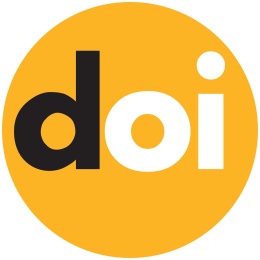
doi:10.3390/en16041760
Publisher Version
Download
Meta
Record Statistics
Record Views
68
Version History
[v1] (Original Submission)
Feb 27, 2023
Verified by curator on
Feb 27, 2023
This Version Number
v1
Citations
Most Recent
This Version
URL Here
https://psecommunity.org/LAPSE:2023.10451
Original Submitter
Auto Uploader for LAPSE
Links to Related Works