LAPSE:2019.1190
Published Article
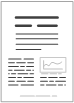
LAPSE:2019.1190
Intelligent Control Strategy for Transient Response of a Variable Geometry Turbocharger System Based on Deep Reinforcement Learning
November 24, 2019
Deep reinforcement learning (DRL) is an area of machine learning that combines a deep learning approach and reinforcement learning (RL). However, there seem to be few studies that analyze the latest DRL algorithms on real-world powertrain control problems. Meanwhile, the boost control of a variable geometry turbocharger (VGT)-equipped diesel engine is difficult mainly due to its strong coupling with an exhaust gas recirculation (EGR) system and large lag, resulting from time delay and hysteresis between the input and output dynamics of the engine’s gas exchange system. In this context, one of the latest model-free DRL algorithms, the deep deterministic policy gradient (DDPG) algorithm, was built in this paper to develop and finally form a strategy to track the target boost pressure under transient driving cycles. Using a fine-tuned proportion integration differentiation (PID) controller as a benchmark, the results show that the control performance based on the proposed DDPG algorithm can achieve a good transient control performance from scratch by autonomously learning the interaction with the environment, without relying on model supervision or complete environment models. In addition, the proposed strategy is able to adapt to the changing environment and hardware aging over time by adaptively tuning the algorithm in a self-learning manner on-line, making it attractive to real plant control problems whose system consistency may not be strictly guaranteed and whose environment may change over time.
Record ID
Keywords
deep deterministic policy gradient, deep reinforcement learning, self-learning, transient response, variable geometry turbocharger
Subject
Suggested Citation
Hu B, Yang J, Li J, Li S, Bai H. Intelligent Control Strategy for Transient Response of a Variable Geometry Turbocharger System Based on Deep Reinforcement Learning. (2019). LAPSE:2019.1190
Author Affiliations
Hu B: Key Laboratory of Advanced Manufacturing Technology for Automobile Parts, Ministry of Education, Chongqing University of Technology, Chongqing 400054, China; State Key Laboratory of Engines, Tianjin University, Tianjin 300072, China [ORCID]
Yang J: Key Laboratory of Advanced Manufacturing Technology for Automobile Parts, Ministry of Education, Chongqing University of Technology, Chongqing 400054, China
Li J: Key Laboratory of Advanced Manufacturing Technology for Automobile Parts, Ministry of Education, Chongqing University of Technology, Chongqing 400054, China
Li S: Key Laboratory of Advanced Manufacturing Technology for Automobile Parts, Ministry of Education, Chongqing University of Technology, Chongqing 400054, China
Bai H: Key Laboratory of Advanced Manufacturing Technology for Automobile Parts, Ministry of Education, Chongqing University of Technology, Chongqing 400054, China
[Login] to see author email addresses.
Yang J: Key Laboratory of Advanced Manufacturing Technology for Automobile Parts, Ministry of Education, Chongqing University of Technology, Chongqing 400054, China
Li J: Key Laboratory of Advanced Manufacturing Technology for Automobile Parts, Ministry of Education, Chongqing University of Technology, Chongqing 400054, China
Li S: Key Laboratory of Advanced Manufacturing Technology for Automobile Parts, Ministry of Education, Chongqing University of Technology, Chongqing 400054, China
Bai H: Key Laboratory of Advanced Manufacturing Technology for Automobile Parts, Ministry of Education, Chongqing University of Technology, Chongqing 400054, China
[Login] to see author email addresses.
Journal Name
Processes
Volume
7
Issue
9
Article Number
E601
Year
2019
Publication Date
2019-09-06
Published Version
ISSN
2227-9717
Version Comments
Original Submission
Other Meta
PII: pr7090601, Publication Type: Journal Article
Record Map
Published Article
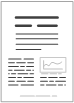
LAPSE:2019.1190
This Record
External Link
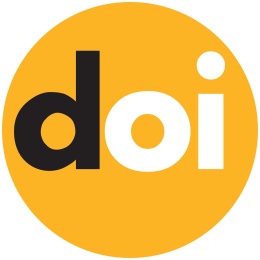
doi:10.3390/pr7090601
Publisher Version
Download
Meta
Record Statistics
Record Views
405
Version History
[v1] (Original Submission)
Nov 24, 2019
Verified by curator on
Nov 24, 2019
This Version Number
v1
Citations
Most Recent
This Version
URL Here
https://psecommunity.org/LAPSE:2019.1190
Original Submitter
Calvin Tsay
Links to Related Works