LAPSE:2024.0916v1
Published Article
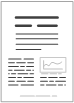
LAPSE:2024.0916v1
Data-Driven Process Monitoring and Fault Diagnosis: A Comprehensive Survey
June 7, 2024
This paper presents a comprehensive review of the historical development, the current state of the art, and prospects of data-driven approaches for industrial process monitoring. The subject covers a vast and diverse range of works, which are compiled and critically evaluated based on the different perspectives they provide. Data-driven modeling techniques are surveyed and categorized into two main groups: multivariate statistics and machine learning. Representative models, namely principal component analysis, partial least squares and artificial neural networks, are detailed in a didactic manner. Topics not typically covered by other reviews, such as process data exploration and treatment, software and benchmarks availability, and real-world industrial implementations, are thoroughly analyzed. Finally, future research perspectives are discussed, covering aspects related to system performance, the significance and usefulness of the approaches, and the development environment. This work aims to be a reference for practitioners and researchers navigating the extensive literature on data-driven industrial process monitoring.
Record ID
Keywords
fault detection and diagnosis, latent variable modeling, Machine Learning, Multivariate Statistics, process monitoring, process systems engineering
Subject
Suggested Citation
Melo A, Câmara MM, Pinto JC. Data-Driven Process Monitoring and Fault Diagnosis: A Comprehensive Survey. (2024). LAPSE:2024.0916v1
Author Affiliations
Melo A: Programa de Engenharia Química/COPPE, Universidade Federal do Rio de Janeiro, Cidade Universitária, Rio de Janeiro CEP 21941-972, Brazil; Centro de Pesquisas Leopoldo Americo Miguez de Mello—CENPES, Petrobras—Petróleo Brasileiro SA, Rio de Janeiro [ORCID]
Câmara MM: Programa de Engenharia Química/COPPE, Universidade Federal do Rio de Janeiro, Cidade Universitária, Rio de Janeiro CEP 21941-972, Brazil; OPTIMATECH Ltda., Cidade Universitária, Rio de Janeiro CEP 21941-614, Brazil [ORCID]
Pinto JC: Programa de Engenharia Química/COPPE, Universidade Federal do Rio de Janeiro, Cidade Universitária, Rio de Janeiro CEP 21941-972, Brazil [ORCID]
Câmara MM: Programa de Engenharia Química/COPPE, Universidade Federal do Rio de Janeiro, Cidade Universitária, Rio de Janeiro CEP 21941-972, Brazil; OPTIMATECH Ltda., Cidade Universitária, Rio de Janeiro CEP 21941-614, Brazil [ORCID]
Pinto JC: Programa de Engenharia Química/COPPE, Universidade Federal do Rio de Janeiro, Cidade Universitária, Rio de Janeiro CEP 21941-972, Brazil [ORCID]
Journal Name
Processes
Volume
12
Issue
2
First Page
251
Year
2024
Publication Date
2024-01-24
Published Version
ISSN
2227-9717
Version Comments
Original Submission
Other Meta
PII: pr12020251, Publication Type: Review
Record Map
Published Article
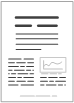
LAPSE:2024.0916v1
This Record
External Link
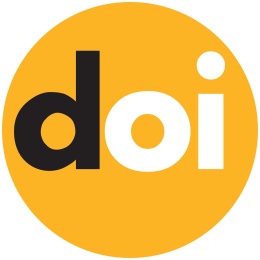
doi:10.3390/pr12020251
Publisher Version
Download
Meta
Record Statistics
Record Views
26
Version History
[v1] (Original Submission)
Jun 7, 2024
Verified by curator on
Jun 7, 2024
This Version Number
v1
Citations
Most Recent
This Version
URL Here
https://psecommunity.org/LAPSE:2024.0916v1
Original Submitter
Auto Uploader for LAPSE
Links to Related Works