LAPSE:2024.0276
Document
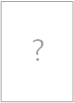
LAPSE:2024.0276
Supplementary Materials to “Learn-To-Design: Reinforcement Learning-Assisted Chemical Process Optimization”
April 8, 2024
This paper proposes an AI-assisted approach aimed at accelerating chemical process design through causal incremental reinforcement learning (CIRL) where an intelligent agent is interacting iteratively with a process simulation environment (e.g., Aspen HYSYS, DWSIM, etc.). The proposed approach is based on an incremental learnable optimizer capable of guiding multi-objective optimization towards optimal design variable configurations, depending on several factors including the problem complexity, selected RL algorithm and hyperparameters tuning. One advantage of this approach is that the agent-simulator interaction significantly reduces the vast search space of design variables, leading to an accelerated and optimized design process. This is a generic causal approach that enables the exploration of new process configurations and provides actionable insights to designers to improve not only the process design but also the design process across various applications. The approach was validated on industrial processes including an absorption-based car-bon capture, considering the economic and technological uncertainties of different capture processes, such as energy price, production cost, and storage capacity. It achieved a cost reduction of up to 5.5% for the designed capture process, after a few iterations, while also providing the de-signer with actionable insights. From a broader perspective, the proposed approach paves the way for accelerating the adoption of decarbonization technologies (CCUS value chains, clean fuel pro-duction, etc.) at a larger scale, thus catalyzing climate change mitigation.
Record ID
Keywords
Artificial Intelligence, Carbon Capture, Machine Learning, Process Design Optimization, Reinforcement Learning, Simulation-based Optimization
Subject
Suggested Citation
Al-Sakkari E, Ragab A, Ali M, Dagdougui H, Boffito DC, Amazouz M. Supplementary Materials to “Learn-To-Design: Reinforcement Learning-Assisted Chemical Process Optimization”. (2024). LAPSE:2024.0276
Author Affiliations
Al-Sakkari E: Polytechnique Montréal
Ragab A*: Polytechnique Montréal
Ali M: Natural Resources Canada
Dagdougui H: Polytechnique Montréal
Boffito DC: Polytechnique Montréal
Amazouz M: Natural Resources Canada
* Corresponding Author
[Login] to see author email addresses.
Ragab A*: Polytechnique Montréal
Ali M: Natural Resources Canada
Dagdougui H: Polytechnique Montréal
Boffito DC: Polytechnique Montréal
Amazouz M: Natural Resources Canada
* Corresponding Author
[Login] to see author email addresses.
Year
2024
Publication Date
2024-07-18
Version Comments
Original Submission
Download
Meta
Record Statistics
Record Views
26
Version History
[v1] (Original Submission)
Apr 8, 2024
Verified by curator on
Apr 11, 2024
This Version Number
v1
Citations
Most Recent
This Version
URL Here
https://psecommunity.org/LAPSE:2024.0276
Original Submitter
aragab