LAPSE:2024.0239
Published Article
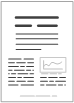
LAPSE:2024.0239
Anomaly Recognition, Diagnosis and Prediction of Massive Data Flow Based on Time-GAN and DBSCAN for Power Dispatching Automation System
February 10, 2024
Existing power anomaly detection is mainly based on analyzing static offline data. But this method takes a long time and has low identification accuracy when detecting timing and frequency anomalies, since this method requires offline screening, classification and preprocessing of the collected data, which is very laborious. Anomaly detection with supervised learning requires a large amount of abnormal data and cannot detect unknown anomalies. So, this paper innovatively proposes the idea of applying Time-series Generative Adversarial Networks (Time-GAN) in a dispatching automation system for the identification, diagnosis and prediction of massive data flow anomalies. First of all, regarding the problem of insufficient abnormal data, we use Time-GAN to generate a large number of reliable datasets for training fault diagnosis models. In addition, Time-GAN can ameliorate the imbalance between various types of data. Secondly, unsupervised learning methods such as Density-Based Spatial Clustering of Applications with Noise (DBSCAN) and K-means are used to detect unknown anomalies that may exist in the power grid. Finally, some supervised learning methods are selected to compare with unsupervised learning methods. Experimental results show that the proposed algorithm has a higher recognition rate of known anomalies than other benchmark algorithms and it can find new unknown anomalies. It lays a good foundation for the safe, stable, high-quality and economical operation of the power grid.
Record ID
Keywords
DBSCAN, fault diagnosis, fault prediction, supervised learning, Time-GAN
Subject
Suggested Citation
Liu W, Lei P, Xu D, Zhu X. Anomaly Recognition, Diagnosis and Prediction of Massive Data Flow Based on Time-GAN and DBSCAN for Power Dispatching Automation System. (2024). LAPSE:2024.0239
Author Affiliations
Liu W: College of Telecommunications and Information Engineering, Nanjing University of Posts and Telecommunications, Nanjing 210003, China [ORCID]
Lei P: College of Telecommunications and Information Engineering, Nanjing University of Posts and Telecommunications, Nanjing 210003, China
Xu D: College of Telecommunications and Information Engineering, Nanjing University of Posts and Telecommunications, Nanjing 210003, China
Zhu X: College of Telecommunications and Information Engineering, Nanjing University of Posts and Telecommunications, Nanjing 210003, China [ORCID]
Lei P: College of Telecommunications and Information Engineering, Nanjing University of Posts and Telecommunications, Nanjing 210003, China
Xu D: College of Telecommunications and Information Engineering, Nanjing University of Posts and Telecommunications, Nanjing 210003, China
Zhu X: College of Telecommunications and Information Engineering, Nanjing University of Posts and Telecommunications, Nanjing 210003, China [ORCID]
Journal Name
Processes
Volume
11
Issue
9
First Page
2782
Year
2023
Publication Date
2023-09-18
Published Version
ISSN
2227-9717
Version Comments
Original Submission
Other Meta
PII: pr11092782, Publication Type: Journal Article
Record Map
Published Article
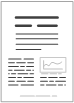
LAPSE:2024.0239
This Record
External Link
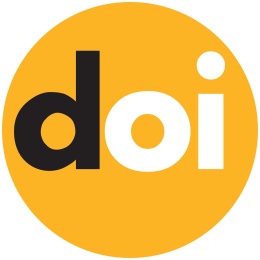
doi:10.3390/pr11092782
Publisher Version
Download
Meta
Record Statistics
Record Views
52
Version History
[v1] (Original Submission)
Feb 10, 2024
Verified by curator on
Feb 10, 2024
This Version Number
v1
Citations
Most Recent
This Version
URL Here
https://psecommunity.org/LAPSE:2024.0239
Original Submitter
Calvin Tsay
Links to Related Works