LAPSE:2024.0068
Published Article
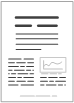
LAPSE:2024.0068
Exploring Bayesian Optimization for Photocatalytic Reduction of CO2
January 12, 2024
The optimization of photocatalysis is complex, as heterogenous catalysis makes its kinetic modeling or design of experiment (DOE) significantly more difficult than homogeneous reactions. On the other hand, Bayesian optimization (BO) has been found to be efficient in the optimization of many complex chemical problems but has rarely been studied in photocatalysis. In this paper, we developed a BO platform and applied it to the optimization of three photocatalytic CO2 reduction systems that have been kinetically modeled in previous studies. Three decision variables, namely, partial pressure of CO2, partial pressure of H2O, and reaction time, were used to optimize the reaction rate. We first compared BO with the traditional DOE methods in the Khalilzadeh and Tan systems and found that the optimized reaction rates predicted by BO were 0.7% and 11.0% higher, respectively, than the best results of optimization by DOE, and were significantly better than the original experimental data, which were 1.9% and 13.6% higher, respectively. In both systems, we also explored the best combination of the surrogate model and acquisition function for BO, and the results showed that the combination of Gaussian processes (GP) and upper confidence bound (UCB) had the most stable search performance. Furthermore, the Thompson system with time dependence was optimized with BO according to the selectivity of CH4. The results showed that the optimized reaction time of BO agreed with the actual experimental data with an error of less than 5%. These results suggest that BO is a more promising alternative to kinetic modeling or traditional DOE in the efficient optimization of photocatalytic reduction.
Record ID
Keywords
Bayesian optimization, design of experiment, Machine Learning, photocatalytic reduction, reaction optimization
Subject
Suggested Citation
Zhang Y, Yang X, Zhang C, Zhang Z, Su A, She YB. Exploring Bayesian Optimization for Photocatalytic Reduction of CO2. (2024). LAPSE:2024.0068
Author Affiliations
Zhang Y: College of Chemical Engineering, Zhejiang University of Technology, Hangzhou 310014, China
Yang X: Key Laboratory of Pharmaceutical Engineering of Zhejiang Province, Collaborative Innovation Center of Yangtze River Delta Region Green Pharmaceuticals, Zhejiang University of Technology, Hangzhou 310014, China
Zhang C: College of Chemical Engineering, Zhejiang University of Technology, Hangzhou 310014, China
Zhang Z: College of Chemical Engineering, Zhejiang University of Technology, Hangzhou 310014, China
Su A: College of Chemical Engineering, Zhejiang University of Technology, Hangzhou 310014, China; Key Laboratory of Pharmaceutical Engineering of Zhejiang Province, Collaborative Innovation Center of Yangtze River Delta Region Green Pharmaceuticals, Zhejiang Un [ORCID]
She YB: College of Chemical Engineering, Zhejiang University of Technology, Hangzhou 310014, China
Yang X: Key Laboratory of Pharmaceutical Engineering of Zhejiang Province, Collaborative Innovation Center of Yangtze River Delta Region Green Pharmaceuticals, Zhejiang University of Technology, Hangzhou 310014, China
Zhang C: College of Chemical Engineering, Zhejiang University of Technology, Hangzhou 310014, China
Zhang Z: College of Chemical Engineering, Zhejiang University of Technology, Hangzhou 310014, China
Su A: College of Chemical Engineering, Zhejiang University of Technology, Hangzhou 310014, China; Key Laboratory of Pharmaceutical Engineering of Zhejiang Province, Collaborative Innovation Center of Yangtze River Delta Region Green Pharmaceuticals, Zhejiang Un [ORCID]
She YB: College of Chemical Engineering, Zhejiang University of Technology, Hangzhou 310014, China
Journal Name
Processes
Volume
11
Issue
9
First Page
2614
Year
2023
Publication Date
2023-09-01
Published Version
ISSN
2227-9717
Version Comments
Original Submission
Other Meta
PII: pr11092614, Publication Type: Journal Article
Record Map
Published Article
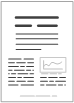
LAPSE:2024.0068
This Record
External Link
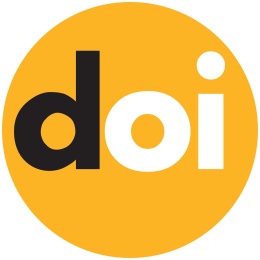
doi:10.3390/pr11092614
Publisher Version
Download
Meta
Record Statistics
Record Views
57
Version History
[v1] (Original Submission)
Jan 12, 2024
Verified by curator on
Jan 12, 2024
This Version Number
v1
Citations
Most Recent
This Version
URL Here
https://psecommunity.org/LAPSE:2024.0068
Original Submitter
Calvin Tsay
Links to Related Works