LAPSE:2024.0059
Published Article
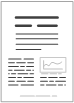
LAPSE:2024.0059
A Sewer Pipeline Defect Detection Method Based on Improved YOLOv5
January 12, 2024
To address the issues of strong subjectivity, low efficiency, and difficulty in on-site model deployment encountered in existing CCTV defect detection of pipelines, this article proposes an object detection model based on an improved YOLOv5s algorithm. Firstly, involution modules and GSConv simplified models are introduced into the backbone network and feature fusion network, respectively, to enhance the detection accuracy. Secondly, a CBAM attention mechanism is integrated to improve the detection accuracy of overlapping targets in complex backgrounds. Finally, knowledge distillation is performed on the improved model to further enhance its accuracy. Experimental results demonstrate that the improved YOLOv5s achieved an mAP@0.5 of 80.5%, which is a 2.4% increase over the baseline, and reduces the parameter and computation volume by 30.1% and 29.4%, respectively, with a detection speed of 75 FPS. This method offers good detection accuracy and robustness while ensuring real-time detection and can be employed in the on-site detection process of sewer pipeline defects.
Record ID
Keywords
attention mechanism, detection of sewer defects, GSConv, improved YOLOv5, involution, knowledge distillation
Subject
Suggested Citation
Wang T, Li Y, Zhai Y, Wang W, Huang R. A Sewer Pipeline Defect Detection Method Based on Improved YOLOv5. (2024). LAPSE:2024.0059
Author Affiliations
Wang T: Henan Key Laboratory of Intelligent Manufacturing of Mechanical Equipment, Zhengzhou University of Light Industry, Zhengzhou 450002, China; College of Mechanical and Electrical Engineering, Zhengzhou University of Light Industry, Zhengzhou 450002, China;
Li Y: Henan Key Laboratory of Intelligent Manufacturing of Mechanical Equipment, Zhengzhou University of Light Industry, Zhengzhou 450002, China; College of Mechanical and Electrical Engineering, Zhengzhou University of Light Industry, Zhengzhou 450002, China
Zhai Y: Henan Key Laboratory of Intelligent Manufacturing of Mechanical Equipment, Zhengzhou University of Light Industry, Zhengzhou 450002, China; College of Mechanical and Electrical Engineering, Zhengzhou University of Light Industry, Zhengzhou 450002, China
Wang W: China Special Equipment Inspection and Research Institute, Beijing 100029, China [ORCID]
Huang R: Henan Key Laboratory of Intelligent Manufacturing of Mechanical Equipment, Zhengzhou University of Light Industry, Zhengzhou 450002, China; College of Mechanical and Electrical Engineering, Zhengzhou University of Light Industry, Zhengzhou 450002, China;
Li Y: Henan Key Laboratory of Intelligent Manufacturing of Mechanical Equipment, Zhengzhou University of Light Industry, Zhengzhou 450002, China; College of Mechanical and Electrical Engineering, Zhengzhou University of Light Industry, Zhengzhou 450002, China
Zhai Y: Henan Key Laboratory of Intelligent Manufacturing of Mechanical Equipment, Zhengzhou University of Light Industry, Zhengzhou 450002, China; College of Mechanical and Electrical Engineering, Zhengzhou University of Light Industry, Zhengzhou 450002, China
Wang W: China Special Equipment Inspection and Research Institute, Beijing 100029, China [ORCID]
Huang R: Henan Key Laboratory of Intelligent Manufacturing of Mechanical Equipment, Zhengzhou University of Light Industry, Zhengzhou 450002, China; College of Mechanical and Electrical Engineering, Zhengzhou University of Light Industry, Zhengzhou 450002, China;
Journal Name
Processes
Volume
11
Issue
8
First Page
2508
Year
2023
Publication Date
2023-08-21
Published Version
ISSN
2227-9717
Version Comments
Original Submission
Other Meta
PII: pr11082508, Publication Type: Journal Article
Record Map
Published Article
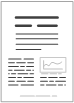
LAPSE:2024.0059
This Record
External Link
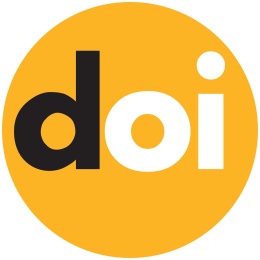
doi:10.3390/pr11082508
Publisher Version
Download
Meta
Record Statistics
Record Views
53
Version History
[v1] (Original Submission)
Jan 12, 2024
Verified by curator on
Jan 12, 2024
This Version Number
v1
Citations
Most Recent
This Version
URL Here
https://psecommunity.org/LAPSE:2024.0059
Original Submitter
Calvin Tsay
Links to Related Works