LAPSE:2023.9759
Published Article
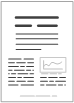
LAPSE:2023.9759
A Review of Auto-Regressive Methods Applications to Short-Term Demand Forecasting in Power Systems
February 27, 2023
The paper conducts a literature review of applications of autoregressive methods to short-term forecasting of power demand. This need is dictated by the advancement of modern forecasting methods and their achievement in good forecasting efficiency in particular. The annual effectiveness of forecasting power demand for the Polish National Power Grid for the next day is approx. 1%; therefore, the main objective of the review is to verify whether it is possible to improve efficiency while maintaining the minimum financial outlays and time-consuming efforts. The methods that fulfil these conditions are autoregressive methods; therefore, the paper focuses on autoregressive methods, which are less time-consuming and, as a result, cheaper in development and applications. The prepared review ranks the forecasting models in terms of the forecasting effectiveness achieved in the literature on the subject, which enables the selection of models that may improve the currently achieved effectiveness of the transmission system operator. Due to the applied approach, a transparent set of forecasting methods and models was obtained, in addition to knowledge about their potential in the context of the needs for short-term forecasting of electricity demand in the national power system. The articles in which the MAPE error was used to assess the quality of short-term forecasts were analyzed. The investigation included 47 articles, several dozen forecasting methods, and 264 forecasting models. The articles date from 1997 and, apart from the autoregressive methods, also include the methods and models that use explanatory variables (non-autoregressive ones). The input data used come from the period 1998−2014. The analysis included 25 power systems located on four continents (Asia, Europe, North America, and Australia) that were published by 44 different research teams. The results of the review show that in the autoregressive methods applied to forecasting short-term power demand, there is a potential to improve forecasting effectiveness in power systems. The most promising prognostic models using the autoregressive approach, based on the review, include Fuzzy Logic, Artificial Neural Networks, Wavelet Artificial Neural Networks, Adaptive Neurofuse Inference Systems, Genetic Algorithms, Fuzzy Regression, and Data Envelope Analysis. These methods make it possible to achieve the efficiency of short-term forecasting of electricity demand with hourly resolution at the level below 1%, which confirms the assumption made by the authors about the potential of autoregressive methods. Other forecasting models, the effectiveness of which is high, may also prove useful in forecasting by electricity system operators. The paper also discusses the classical methods of Artificial Intelligence, Data Mining, Big Data, and the state of research in short-term power demand forecasting in power systems using autoregressive and non-autoregressive methods and models.
Record ID
Keywords
artificial intelligence methods, autoregressive forecasting methods, Big Data, classical forecasting methods, Data Mining, electrical power demand, Machine Learning, power systems, short-term forecasting
Subject
Suggested Citation
Czapaj R, Kamiński J, Sołtysik M. A Review of Auto-Regressive Methods Applications to Short-Term Demand Forecasting in Power Systems. (2023). LAPSE:2023.9759
Author Affiliations
Czapaj R: PSE Innowacje Sp.z o.o., Al. Jerozolimskie 132 a, 02-305 Warsaw, Poland
Kamiński J: Mineral and Energy Economy Research Institute of the Polish Academy of Sciences, The Department of Policy and Strategic Research, The Division of Energy Economics, Wybickiego 7A, 31-261 Kraków, Poland [ORCID]
Sołtysik M: Faculty of Electrical Engineering, Częstochowa University of Technology, Armii Krajowej 17, 42-200 Częstochowa, Poland [ORCID]
Kamiński J: Mineral and Energy Economy Research Institute of the Polish Academy of Sciences, The Department of Policy and Strategic Research, The Division of Energy Economics, Wybickiego 7A, 31-261 Kraków, Poland [ORCID]
Sołtysik M: Faculty of Electrical Engineering, Częstochowa University of Technology, Armii Krajowej 17, 42-200 Częstochowa, Poland [ORCID]
Journal Name
Energies
Volume
15
Issue
18
First Page
6729
Year
2022
Publication Date
2022-09-14
Published Version
ISSN
1996-1073
Version Comments
Original Submission
Other Meta
PII: en15186729, Publication Type: Review
Record Map
Published Article
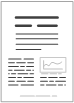
LAPSE:2023.9759
This Record
External Link
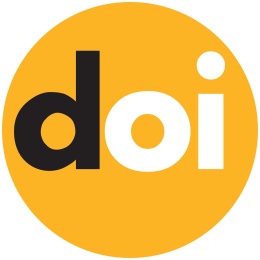
doi:10.3390/en15186729
Publisher Version
Download
Meta
Record Statistics
Record Views
79
Version History
[v1] (Original Submission)
Feb 27, 2023
Verified by curator on
Feb 27, 2023
This Version Number
v1
Citations
Most Recent
This Version
URL Here
https://psecommunity.org/LAPSE:2023.9759
Original Submitter
Auto Uploader for LAPSE
Links to Related Works