LAPSE:2023.8758
Published Article
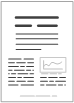
LAPSE:2023.8758
A Filter-Based Feature-Engineering-Assisted SVC Fault Classification for SCIM at Minor-Load Conditions
February 24, 2023
In most manufacturing industries, squirrel cage induction motors (SCIMs) are essential due to their robust nature, high torque generation, and low maintenance costs, so their failure often times affects productivity, profitability, reliability, etc. While various research studies presented techniques for addressing most of these machines’ prevailing issues, fault detection in cases of low slip or, low load, and no loading conditions for motor current signature analysis still remains a great concern. When compared to the impact on the machine at full load conditions, fault detection at low load conditions helps mitigate the impact of the damage on SCIM and reduces maintenance costs. Using stator current data from the SCIM’s direct online starter method, this study presents a feature engineering-aided fault classification method for SCIM at minor-load conditions based on a filter approach using the support vector classification (SVC) algorithm as the classifier. This method leverages the loop-hole of the Fourier Transform at minor-load conditions by harnessing the uniqueness of the Hilbert Transform (HT) to present a methodology that combines different feature engineering technologies to excite, extract, and select 10 discriminant information using a filter-based approach as the selection tool for fault classification. With the selected features, the SVC performed exceptionally well, with a significant diagnostic performance accuracy of 97.32%. Further testing with other well-known robust classifiers such as decision tree (DT), random forest (RF), k-nearest neighbor (KNN), gradient boost classifier (GBC), stochastic gradient descent (SGD), and global assessment metrics revealed that the SVC is reliable in terms of accuracy and computation speeds.
Record ID
Keywords
fault diagnosis, feature engineering, Hilbert transform, Machine Learning, squirrel cage induction motor, support vector classifier
Subject
Suggested Citation
Okwuosa CN, Hur JW. A Filter-Based Feature-Engineering-Assisted SVC Fault Classification for SCIM at Minor-Load Conditions. (2023). LAPSE:2023.8758
Author Affiliations
Okwuosa CN: Department of Mechanical Engineering (Department of Aeronautics, Mechanical and Electronic Convergence Engineering), Kumoh National Institute of Technology, 61 Daehak-ro, Gumi-si 39177, Gyeonsang-buk-do, Korea [ORCID]
Hur JW: Department of Mechanical Engineering (Department of Aeronautics, Mechanical and Electronic Convergence Engineering), Kumoh National Institute of Technology, 61 Daehak-ro, Gumi-si 39177, Gyeonsang-buk-do, Korea
Hur JW: Department of Mechanical Engineering (Department of Aeronautics, Mechanical and Electronic Convergence Engineering), Kumoh National Institute of Technology, 61 Daehak-ro, Gumi-si 39177, Gyeonsang-buk-do, Korea
Journal Name
Energies
Volume
15
Issue
20
First Page
7597
Year
2022
Publication Date
2022-10-14
Published Version
ISSN
1996-1073
Version Comments
Original Submission
Other Meta
PII: en15207597, Publication Type: Journal Article
Record Map
Published Article
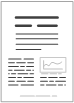
LAPSE:2023.8758
This Record
External Link
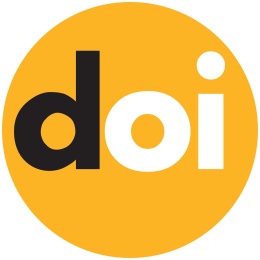
doi:10.3390/en15207597
Publisher Version
Download
Meta
Record Statistics
Record Views
89
Version History
[v1] (Original Submission)
Feb 24, 2023
Verified by curator on
Feb 24, 2023
This Version Number
v1
Citations
Most Recent
This Version
URL Here
https://psecommunity.org/LAPSE:2023.8758
Original Submitter
Auto Uploader for LAPSE
Links to Related Works